Sub-kilometer Precipitation Datasets for Snowpack and Glacier Modeling in Alpine Terrain
- 1Univ. Grenoble Alpes, Université de Toulouse, Météo-France, CNRS, CNRM, Centre d'Études de la Neige, Grenoble, France
- 2Centre for Hydrology, University of Saskatchewan, Saskatoon, SK, Canada
- 3Univ. Grenoble Alpes, CNRS, IRD, Grenoble INP, IGE, Grenoble, France
- 4Université de Toulouse, Météo France, CNRS, CNRM, Toulouse, France
- 5WSL Institute for Snow and Avalanche Research SLF, Davos, Switzerland
- 6Centro de Estudios Avanzados en Zonas Áridas, Universidad de La Serena (ULS), La Serena, Chile
- 7Université Grenoble Alpes, Irstea, UR ETGR, Grenoble, France
Capturing the spatial and temporal variability of precipitation at fine scale is necessary for high-resolution modeling of snowpack and glacier mass balance in alpine terrain. In this study, we assess the impact of three sub-kilometer precipitation datasets on distributed simulations of snowpack and glacier mass balance with the detailed snowpack model Crocus for winter 2011–2012. The different precipitation datasets at 500-m grid spacing over the northern and central French Alps are coming from (i) the SAFRAN reanalysis specially developed for alpine terrain interpolated at 500-m grid spacing, (ii) the numerical weather prediction (NWP) system AROME at 2.5-km resolution downscaled with a precipitation-elevation adjustment factor, and (iii) a version of AROME at 500-m grid spacing. The spatial patterns of seasonal snowfall are first analyzed for the different precipitation datasets. Large differences between SAFRAN and the two versions of AROME are found at high-altitude and in regions of strong orographic precipitation enhancement. Results of Crocus snowpack simulations are then evaluated against (i) point measurements of snow depth, (ii) maps of snow covered areas retrieved from optical satellite data (MODIS) and (iii) field measurements of winter accumulation of six glaciers. The two versions of AROME lead to an overestimation of snow depth and snow-covered area, which are substantially improved by SAFRAN. However, all the precipitation datasets lead to an underestimation of snow depth increase at the daily scale and cumulated over the season, with AROME 500 m providing the best performances at the seasonal scale. The low correlation found between the biases in snow depth and in cumulated snow depth increase illustrates that total snow depth has a limited significance for the evaluation of precipitation datasets. Measurements of glacier winter mass balance showed a systematic underestimation of high-elevation snow accumulation with SAFRAN. The two versions of AROME overestimate the winter mass balance at four glaciers and produce nearly unbiased estimations for two of them. Our study illustrates the need for improvements in the precipitation field from high-resolution NWP systems for snow and glacier modeling in alpine terrain.
1. Introduction
Accurate estimation of winter precipitation stored as snow in mountainous terrain is critical for many applications. For hydrology, mountain snowpacks play a crucial role in providing spring and summer water resources for multiple purposes in different regions of the world (e.g., Viviroli et al., 2007; Mankin et al., 2015). The spatial and temporal evolution of avalanche hazard is also strongly impacted by snow accumulation and the changes in the physical properties of snow on the ground (e.g., Schweizer et al., 2003). Winter snow accumulation exerts also a strong control on the annual mass balance of mountain glaciers (Cuffey and Paterson, 2010; Réveillet et al., 2018; Roth et al., 2018). Finally, errors in the estimation of winter precipitation have the largest impact on snowpack modeling uncertainties (Raleigh et al., 2015).
Winter precipitation in mountainous terrain presents a large spatial and temporal variability influenced by topography at different scales (Mott et al., 2018). At the mountain-range scale, precipitation patterns are mainly driven by orographic precipitation (Roe, 2005; Houze, 2012). The forced ascent of air masses over topography leads to condensation and increased precipitation on the windward side relative to the leeward side of mountain ranges. Changes in the synoptic and local conditions also affect the snowfall limit and the partition between liquid and solid precipitation (Unterstrasser and Zängl, 2006). At the local scale, microphysical processes, such as the seeder-feeder mechanism (Mott et al., 2014) and preferential deposition of snowfall (Lehning et al., 2008) enhance the spatial variability of solid precipitation (Gerber et al., 2019). This multi-scale variability represents a challenge in obtaining reliable precipitation dataset in mountainous terrain.
Networks of gauges measuring precipitation in mountainous terrain are usually scarce so that interpolation techniques taking into account topography have been developed to derive spatially-continuous precipitation datasets. Thornton et al. (1997) proposed a method relying on a precipitation adjustment function depending only on elevation. This method is used to produce the Daymet precipitation dataset at 1-km grid spacing over North America. Other methods were introduced to better account for the influence of synoptic conditions on the relationship between precipitation and elevation used in the interpolation. Over the French mountains, Durand et al. (1993, 2009) developed the precipitation analysis system SAFRAN that combines gauge measurements with climatological gradients of precipitation computed for seven weather regimes. Outputs of SAFRAN are available per elevation-steps for areas of climatological homogeneity known as massifs. Over the same mountains, Gottardi et al. (2012) also used an interpolation method with a classification into weather patterns in their 1-km precipitation dataset. In the United States, Daly et al. (1994, 2008) developed the parameter-elevation regressions on independent slopes model (PRISM) using empirical relationships to account for the influence of elevation, windward and leeward slopes and coastal proximity. PRISM data are available at 4 and 0.8 km grid spacing over the United States. These gauge-based products suffer from limitations in areas where few data are available, for example at high-elevation (e.g., Gerbaux et al., 2005; Gutmann et al., 2012; Silverman et al., 2013; Henn et al., 2018). The measurements of snowfall amount with gauge is also affected by wind-undercatch (e.g., Rasmussen et al., 2012; Kochendorfer et al., 2018) which limits the final quality of the precipitation datasets (Adam and Lettenmaier, 2003).
Precipitation datasets derived from high-resolution atmospheric models constitute an alternative to gauge-based products. They can be obtained from continuous integrations of Regional Climate Models (RCM) or from the combinations of successive forecasts of Numerical Weather Prediction (NWP) systems. Ikeda et al. (2010) and Rasmussen et al. (2011) used the Weather Research and Forecasting (WRF) model (Skamarock and Klemp, 2008) in RCM mode to simulate seasonal snowfall over Colorado. They showed that a minimum grid spacing of 6 km is required to capture topographically-induced vertical motions and resulting snowfall. These results were then confirmed with different RCMs across several mountain ranges of the world: in the United States (Hughes et al., 2017; Jing et al., 2017), in Nepalese Himalaya (Collier and Immerzeel, 2015) and in the European Alps (Ban et al., 2014). Recently, Bonekamp et al. (2018) have shown the potential of WRF at 500-m grid spacing to capture the spatial variability of winter precipitation in Nepalese Himalaya. High-resolution NWP systems at kilometer scale have also been used to generate high-resolution atmospheric forcing for snowpack simulations in alpine terrain (Horton et al., 2015; Quéno et al., 2016; Vionnet et al., 2016; Luijting et al., 2018). These systems benefit from advanced data assimilation systems (e.g., Brousseau et al., 2008) but are still subject to analysis and forecast errors impacting simulated precipitation and the resulting snowpack simulations. Furthermore, they do not assimilate precipitation gauge measurements. Therefore, outputs of NWP systems can be combined with gauge measurements to provide high-resolution precipitation analysis (Soci et al., 2016; Fortin et al., 2018) but these products suffer from the same limitations as the gauge-based products in mountainous terrain (Schirmer and Jamieson, 2015).
In the French mountains, Vionnet et al. (2016) and Quéno et al. (2016) used the NWP system AROME at 2.5 km grid spacing (Seity et al., 2011) to drive snowpack simulations with the detailed snowpack model Crocus (Brun et al., 1992; Vionnet et al., 2012). They showed that AROME captured mesoscale orographic processes leading to a more realistic regional snowpack variability compared to simulations driven by the SAFRAN meteorological analysis system. AROME-Crocus brought also improvements in terms of simulated daily snow accumulation (larger than 10 cm per day) compared to SAFRAN-Crocus due partially to the absence of undercatch correction in SAFRAN. However, AROME-Crocus led to an overall overestimation of snow depth due to (i) locally overestimated orographic precipitation enhancement, (ii) biases in radiative forcing (iii) non-simulated wind-induced snow erosion and (iv) underestimation of snow compaction (Quéno et al., 2016, 2018). These results were obtained with a version of AROME running at 2.5 km grid-spacing whereas the operational version of AROME is currently running at 1.3 km (Brousseau et al., 2016) and a version at 500 m is used in support of airport operations (Hagelin et al., 2014). This sub-kilometer version presents an even larger interest in mountainous terrain to better predict local terrain-induced phenomena and to drive snowpack simulations at sufficient resolution to explicitly capture part of the variability of slope and aspect influencing snow evolution (Revuelto et al., 2018).
The main objective of this study is to assess the ability of AROME at 500-m grid spacing for snow and glacier mass balance modeling in the French Alps. Snowpack simulations were carried out at 500-m grid spacing over the northern and central French Alps for winter 2011/2012. This paper places a specific emphasis on the importance of the precipitation forcing for sub-kilometer snowpack simulations. It compares snowpack simulations driven by AROME 500 m with simulations where the precipitation forcing is obtained from (i) AROME 2.5 km to assess the role of model resolution and (ii) the SAFRAN analysis system taken as a reference precipitation dataset in the French mountains. Results of snowpack simulations are evaluated against (i) ground-based measurements of snow depth at 87 stations, (ii) maps of snow covered areas retrieved from optical satellite data (MODIS) and (iii) measurements of winter snow accumulation at six glaciers. The paper is organized as follows: section 2 describes the study area, the different precipitation datasets, the numerical modeling strategy and the verification method. Results are then detailed in section 3. The precipitation datasets are first compared and results of snowpack simulations are then presented. Section 4 contains a discussion about the main results of this study. Finally, section 5 summarizes the results and offers concluding remarks.
2. Methodology
2.1. Study Area and Period
This study focuses on the northern and central French Alps over a domain covering 155 km (West-East) × 200 km (North-South). Figure 1 details the topography of the region at 500-m grid spacing. The highest summits (above 4,200 m) are located in the Mont Blanc mountain range (area 3 on Figure 1) and areas above 2,000 m are widely spread across the domain which makes it relevant to study winter precipitation dataset and related snowpack evolution in alpine regions. Several glaciers are also located in the study area, mainly in the Mont Blanc, Vanoise, Grandes Rousses, Oisans, and Pelvoux mountain ranges as shown by the glacier mask of Gardent et al. (2014) reported in red on Figure 1.
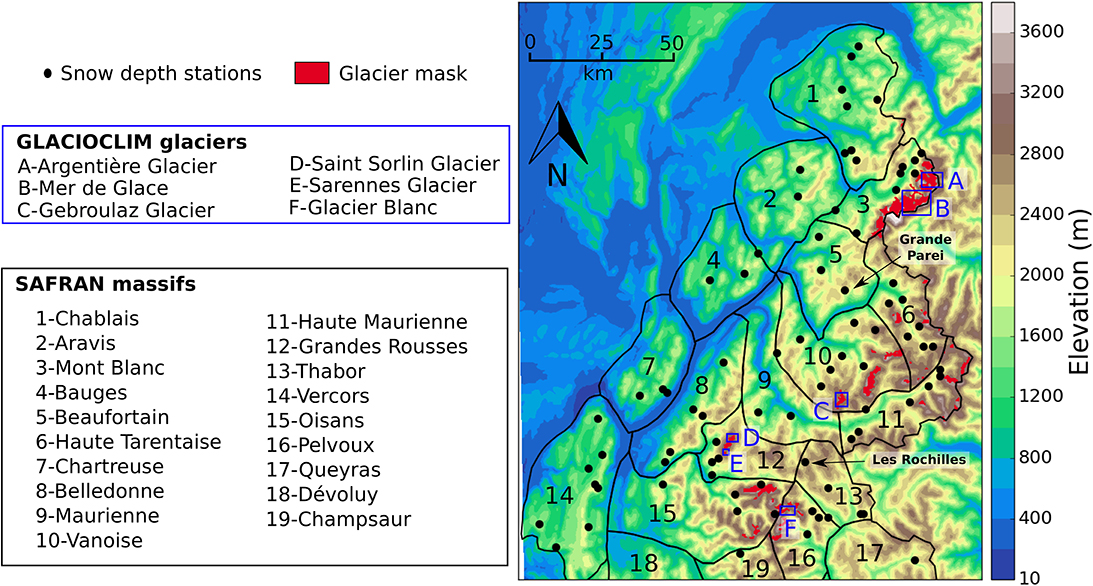
Figure 1. Map of the simulation domain showing the topography at 500-m grid spacing. Black dots show the location of stations measuring snow depth (87 in total). The glacier mask (see text for more details) appears in red (only available in France) and glaciers monitored by the GLACIOCLIM observatory (blue boxes) are listed. Contours (black lines) and names of the SAFRAN massifs are also given on the map.
The study period goes from September 1st 2011 to June 30th 2012. This relatively short period was constrained by the high computational request of AROME 500 m. Winter 2011/2012 has been characterized by dry conditions with little snow accumulation up to high altitude until the beginning of December. Then, large precipitation amounts in December and January led to snow accumulation above average above 2,000 m at the end of January. During this period, precipitation amounts tended to be larger in the northern French Alps than in the central and southern parts. February was characterized by dry and very cold conditions followed by warmer conditions in March. The final substantial snowfall were observed above 1,500 m at the beginning of April.
2.2. Precipitation Dataset and Atmospheric Forcing
In this study, we assess the impact of three precipitation datasets on distributed simulations of snowpack and glacier winter mass balance at 500-m grid spacing in the northern and central French Alps (Figure 1). These hourly datasets are described below. They cover the period from September 1st 2011 to June 30th 2012.
2.2.1. AROME NWP System
AROME is the NWP system used by Météo France to provide short-range forecast at high resolution (2.5 km from 2008 to 2015, 1.3 km since then) (Seity et al., 2011; Brousseau et al., 2016). AROME is particularly relevant to represent small-scale processes in mountainous terrain including orographic precipitation and valley winds. In this paper, two methods are considered to obtain hourly precipitation dataset at 500-m grid spacing from AROME.
In the first method, daily forecasts at 2.5 km issued at the 0 UTC analysis time were considered as in Quéno et al. (2016) and Vionnet et al. (2016). Successive hourly precipitation forecasts (solid + liquid) at 6–29 h lead time were extracted to avoid precipitation spin up and combined together to generate a continuous precipitation dataset at 2.5 km over the domain of study (Figure 1). This 2.5-km precipitation dataset was then downscaled to the final 500-m grid using the precipitation adjustment function proposed by Liston and Elder (2006):
where P500m (in mm) and z500m (in km) are the resulting total precipitation and the elevation on the 500-m grid, respectively, while P2.5km and z2.5km are the total precipitation and the elevation from the 2.5 km grid bilinearily interpolated on the 500 m grid. χ is a precipitation-elevation adjustment factor that depends on the season (Thornton et al., 1997; Liston and Elder, 2006). In our study, χ is set to 0.30 km−1, the average of the monthly values for the period from September to June reported in Liston and Elder (2006).
In the second method, a version of AROME at 500 m has been used to dynamically downscale AROME forecast from 2.5 km to 500 m over our region of interest. At 500 m, AROME uses similar physical parameterizations and dynamical options as the operational configuration at 2.5 km (Seity et al., 2011) with the exception of a more stable temporal scheme (predictor-corrector). AROME at 500 m differs in terms of vertical discretization (60 levels in the 2.5-km version, 90 in the 500-m version). AROME at 500 m ran over a domain covering 250 km (West-East) × 250 km (North-South) and centered over the region of study. For each day from September 1st 2011 to June 30th 2012, a 30-h forecast issued at 00 UTC was generated with AROME 500-m with initial and lateral boundary conditions taken from the operational version at 2.5 km. As in the first method, successive forecasts of liquid and solid precipitation at 6–29 h lead time were then extracted and combined together to generate a continuous precipitation dataset at 500-m grid spacing.
2.2.2. SAFRAN Reanalysis
SAFRAN (Systeme d'Analyse Fournissant des Renseignements Atmosphériques à la Neige; Analysis System Providing Atmospheric Information to Snow) (Durand et al., 1993, 2009) is a meteorological reanalysis system specially developed for alpine terrain. SAFRAN provides hourly meteorological information in 300-m elevation steps for 23 areas of the French Alps known as massifs (Figure 1). These different massifs were defined for their climatological homogeneity (Durand et al., 1993). The precipitation analysis in SAFRAN relies on observations from automatic stations and manual stations across the different massifs (~300 stations in wintertime covering the altitude range 500–2,500 m). For each massif, SAFRAN uses an objective analysis method based on optimal interpolation to combine these observations with a climatological vertical profile of precipitation computed for seven weather regimes. In our study, SAFRAN total precipitation was interpolated on the 500-m grid depending on the elevation and the massif of each grid cell of the domain as in Vionnet et al. (2016). SAFRAN data are only available within the limits of the massifs shown on Figure 1, at elevations higher than 600 m.
2.2.3. Atmospheric Forcing for Snowpack Simulations
The three precipitation datasets were integrated in different sets of atmospheric forcing for the detailed snowpack model SURFEX/Crocus (Brun et al., 1989, 1992; Vionnet et al., 2012). In addition to solid and liquid precipitation, Crocus requires the following meteorological forcing: air temperature and specific humidity at a given level (generally 2 m), wind speed at a given level (generally 10 m) and surface incoming longwave and shortwave radiation. These fields were taken from the AROME forecast at 500-m grid spacing using the same method as in Quéno et al. (2016) and Vionnet et al. (2016): successive forecasts from the 00 UTC analysis time at 6–29 h lead time were combined together to generate a continuous atmospheric forcing. These forcing were combined with the precipitation dataset to generate three atmospheric forcing:
• ARO_0p5: the precipitation are taken from AROME at 500-m grid spacing
• ARO_2p5D: the precipitation are taken from AROME 2.5 km downscaled on the 500-m grid.
• SAFRAN: the precipitation are taken from SAFRAN interpolated on the 500-m grid.
For the forcing datasets SAFRAN and ARO_2p5D, the separation between solid and liquid precipitation is based on a threshold of AROME-500 m air temperature fixed at 1°C. In ARO_0p5, the phase separation is directly provided by the cloud microphysical scheme running in AROME 500 m.
2.3. Snowpack Simulations
Snowpack simulations driven by the different atmospheric forcing described in section 2.2.3 were performed using the detailed snowpack model SURFEX/Crocus (Brun et al., 1989, 1992; Vionnet et al., 2012) coupled with the ISBA land surface model within the SURFEX interface (Decharme et al., 2011; Masson et al., 2013). The default physical options are used for all processes as defined in Lafaysse et al. (2017). For reproducibility of results, the code version used in this study is tagged as Vionnet_Frontiers2019 in the SURFEX repository (https://opensource.umr-cnrm.fr/projects/surfex). Distributed snowpack simulations were carried out over the 500-m grid from September 1st 2011 to June 30th 2012. Effects of terrain slope and aspect on incoming shortwave radiation as well as terrain shading due to the surrounding topography were included as in Revuelto et al. (2016).
The initial soil state was provided by a 1-year spin up using AROME operational forcing at 2.5 km interpolated over the 500-m grid. A specific initialization was used over glacierized areas to ensure glacier presence during the whole simulation period as in Revuelto et al. (2018). The six deepest layers in Crocus were used to initialize the ice profile over the grid points covered by glaciers. They were initialized with a density value of 917 kg m−3 and a temperature of 273.16 K. Their initial thickness was set to 0.01, 0.05, 0.25, 1.25, 6.25, 31.25 m, resulting in a total initial ice thickness of 39.06 m. The extent of glacierized areas was based on the glacier inventory of Gardent et al. (2014) and is shown on Figure 1. SURFEX/Crocus ran assuming no interaction between snow and vegetation as in Vionnet et al. (2016) and Quéno et al. (2016) which limits the reliability of the snowpack simulations in forested areas.
2.4. Data and Evaluation Methods
2.4.1. Snow Depth Data
Snow depth measurements were taken from two sources: (i) a network of automatic stations measuring hourly snow depth using ultra-sonic sensors and (ii) a network of manual stations located in ski resorts where an operator measures snow depth twice a day on a fixed snow stake from the opening to the closing dates of the ski resorts (typically from early December to late March/early April). Stations were selected following several criteria. First, the stations had to be within the boundaries of the SAFRAN massifs (Figure 1). Then, only stations where the difference between the model elevation and the actual terrain elevation at the location of the station is lower than 150 m in absolute value were kept for model verification. Similar criteria have been previously applied in studies evaluating distributed snowpack simulations in the French Alps and Pyrenees (Quéno et al., 2016; Vionnet et al., 2016). The threshold on elevation difference is mainly applied to limit error in snowpack simulation resulting from error in precipitation phase. Overall, 87 stations measuring daily snow depth were available for model evaluation in our study (Figure 1). The bias and mean absolute error between the elevation of the stations and the corresponding points on the 500-m grid are −18 and 63 m, respectively. The stations cover an altitudinal range from 631 to 3,000 m with a median elevation of 1,700 m (upper quartile: 2,030 m; lower quartile: 1,408 m). Only five stations are located above 2,500 m.
Snow depth observations available daily at 06 UTC were used to derive error statistics [bias and root mean square error (RMSE)] of the simulated daily snow depth for the snowpack simulations driven by different atmospheric forcings. These errors statistics were computed from October 1st 2011 to June 30th 2012 and for three sub-periods: (i) early snow-season (October, November, and December; OND), (ii) mid-winter (January, February, and March; JFM) and (iii) late season (April, May, June; AMJ). Three elevation bands were also considered to compute the scores: (i) 600–1,500 m (25 stations), (ii) 1,500–2,000 m (39 stations) and (iii) 2,000–3,000 m (23 stations). None of the selected stations was located above 3,000 m. Despite their altitudinal and spatial coverage (Figure 1), these point measurements are affected by a limited spatial representativeness (Grünewald and Lehning, 2015) that can impact the evaluation of gridded snowpack simulations. In particular, the local slope at the station location may differ from the slope resolved at 500-m grid spacing. In addition, the evolution of the snowpack at these locations is potentially influenced by wind-induced snow transport and preferential deposition of snowfall (e.g., Mott et al., 2018) that are not simulated in our Crocus experiments and that can affect the evaluation of model performances (Quéno et al., 2016).
Daily snow depth variations, ΔHS, were also considered since they provide additional information on the processes responsible for errors in simulated snow depth (Quéno et al., 2016), in particular the quality of the precipitation forcing (Schirmer and Jamieson, 2015). They were analyzed in two ways. First, observed and simulated ΔHS were computed at each station. The Equitable Threat Score (ETS, Supplement Section 1) was then computed to quantify the skill of each snowpack simulation for different thresholds of ΔHS (1, 5, 10, 20, 40, and 60 cm). Cumulated ΔHS by category were also computed as in Schirmer and Jamieson (2015) and Quéno et al. (2016). This analysis was carried out for several elevation bands (600–1,500, 1,500–2,000, 2,000–3,000 m) to identify if the different snowpack simulations led to under- or over-estimation of ΔHS for the different categories. In addition, cumulative sums of the positive daily snow depth variations, ΣΔHS+, were computed at each station with a snow depth record covering the full season. 26 stations were used. Observed and simulated ΣΔHS+ at the end of the winter were finally compared. Currier et al. (2017) used such method when evaluating different datasets of solid precipitation in the Olympic Mountains (USA).
2.4.2. MODIS Snow Cover Images
MODIS fractional snow cover images were used to evaluate the spatial variability of the simulated snow cover. The MOD10A1 product Collection 6 (Riggs et al., 2017) distributed by the NSIDC (National Snow and Ice Data Center) was selected in our study due to its spatial (500 m) and temporal (daily) resolution. Masson et al. (2018) have also shown significant improvements of the MOD10A1 product Collection 6 compared to the older Collection 5 in the French Alps. As in Masson et al. (2018), MOD10A1 Collection 6 maps of Normalized Difference Snow Index were converted into maps of snow cover fraction following the method proposed by Salomonson and Appel (2004).
Maps of snow cover fraction from MODIS were firstly used to visually compare the geographical extent of observed and simulated snow cover at different stages of the snow season. For this purpose, binary maps of simulated snow cover were obtained using a Snow Water Equivalent (SWE) threshold of 20 kg m−2 as in Quéno et al. (2016). Second, we quantitatively compared the temporal evolution of observed and simulated snow cover area (SCA). In this case, a threshold value of 50% on the snow cover fraction was applied to derive binary maps of snow cover from MODIS. The comparison of SCA was carried out for the region within the limits of the SAFRAN massifs (Figure 1) and for several elevations bands within these limits (600–1,500, 1,500–2,000, 2,000–2,500 m, above 2,500 m). We also evaluated the spatial similarity between the observed and simulated SCA using the Jaccard index, J, as in Quéno et al. (2016) and Revuelto et al. (2018). J is the ratio between the intersection between the observed (O) and the simulated (S) snow cover area and their union:
J values range from 0 to 1, with the value of 1 representing a perfect match between the observed and simulated SCA.
MODIS snow cover data are affected by several limitations (e.g., Parajka et al., 2012). Cloud obstruction and terrain shadowing reduce the availability of data. In addition, the accuracy of MOD10A1 is lower in forested area. This is also the case for our snowpack simulations that did not account for snow/canopy interactions. Therefore, a careful selection was applied to select days and pixels used to compute SCA and J. First, only days where the total cloud cover was below 15% were considered for the analysis. Then, for each selected day, pixels that were covered by clouds or those where MODIS gave no data were removed from the analysis. Finally, pixels covered by forest were also removed. Details on the forest mask are given in Supplement Section 2 and in Figure S1. Observed and simulated SCA for the remaining valid pixels were compared.
2.4.3. Glacier Winter Surface Mass Balance
Winter surface mass balance (WSMB) data from six glaciers across the French Alps were used. Their location is shown on Figure 1 and Table 1 depicts their main characteristics. These glaciers are part of the GLACIOCLIM observatory (Six and Vincent, 2014). The surface mass balance (meaning the sum of winter surface mass balance and summer surface mass balance, expressed in kg m−2) is currently retrieved at distinct points over the entire glacier using the glaciological method (Cuffey and Paterson, 2010). WSMB is measured using drilling cores and density measurements over accumulation and ablation area at the end of the snow accumulation period (late April-beginning of May). The uncertainties at each drilling cores are evaluated at ~ ±200 kg m−2 (Thibert et al., 2008). The summer surface mass balance is measured at the same location as WSMB, using stakes inserted in snow and ice. Measurements are collected at several dates throughout the summer until the beginning of October considered as the beginning of the snow accumulation season. Measurements points over these glaciers cover on average higher altitudes (between 2,000 and 3,500 m, depending on the glacier) than the conventional stations measuring snow depth.
Values of simulated WSMB were obtained for each glacier and each Crocus simulation. Simulated WSMB is computed as the difference of simulated SWE between the observation dates (beginning and end of the snow accumulation period) for the simulation pixels in which drilling cores are located. WSMB simulated by Crocus includes the accumulation of snowfall and mass loss due to surface sublimation and melt, that can potentially occurs during the accumulation season. Error statistics (bias and RMSE) were derived for each Crocus simulation and each glacier. For the Argentière and Mer de Glace glaciers, error statistics were also computed for drilling cores above 2,600 m, since these points are less prone to snow melt during the accumulation season than the drilling cores located at lower elevation. We finally compared simulated and observed WSMB as a function of elevation.
Similar to snow depth measurements (section 2.4.1), the comparison between gridded simulations and drilling cores measurements is also affected by the limited representativeness of the measurements. In addition, preferential deposition of snowfall and gravitational snow transport influence snow accumulation over glaciers (e.g., Dadic et al., 2010; Helfricht et al., 2015) and are not represented in the Crocus simulations. These processes can create regions of preferential snow accumulation over glaciers relative to surrounding non-glacierized area at similar elevation (Gascoin et al., 2013) and differences of snow accumulation between adjacent glaciers (Machguth et al., 2006). None of the drilling cores used in this study are located in areas of glacier fed directly by avalanches.
3. Results
3.1. Comparison of Precipitation Datasets
Figure 2 presents the maps of accumulated snowfall from September 1st 2011 to June 30th 2012 for the different precipitation datasets as well as the difference maps between the datasets. On these maps, SAFRAN data are only presented within the limits of the massifs since they are not available outside. In addition, Figure 3 gives the average annual snowfall for each massif and each dataset. For all datasets, total snowfall increases from the massifs located on the northwest foothills of the French Alps (Chablais, Aravis, Bauges, Chartreuse, Vercors; Figure 1) toward the inner and higher massifs, such as Mont Blanc, Vanoise, Haute Tarentaise, Belledonne, or Grandes Rousses. In particular, the maxima of seasonal snowfall for each dataset is obtained for the Mont Blanc massif characterized by the highest elevations in the French Alps (Figure 1). Cumulated snowfalls tend to be lower in the southeast part of our region of interest, for example over the Queyras massif.
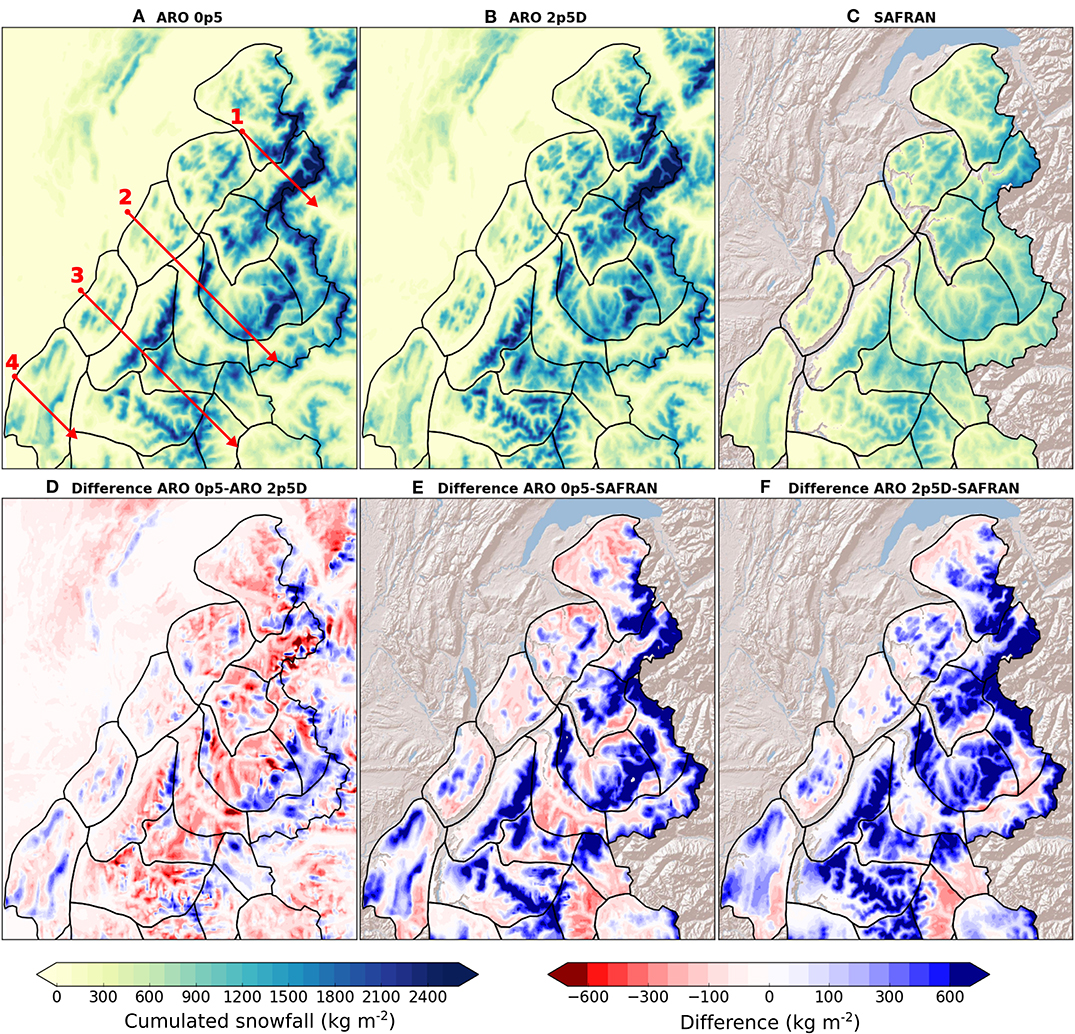
Figure 2. Total snowfall from September 1st 2011 to June 30th 2012 for the different precipitation datasets: (A) AROME 500 m (ARO_0p5), (B) downscaled AROME 2.5 km (ARO_2p5D), and (C) SAFRAN. Maps (D–F): differences between the datasets. SAFRAN data are only available within the limits of the massifs (black lines). Map (A) shows the location of the four transects detailed on Figure 4.
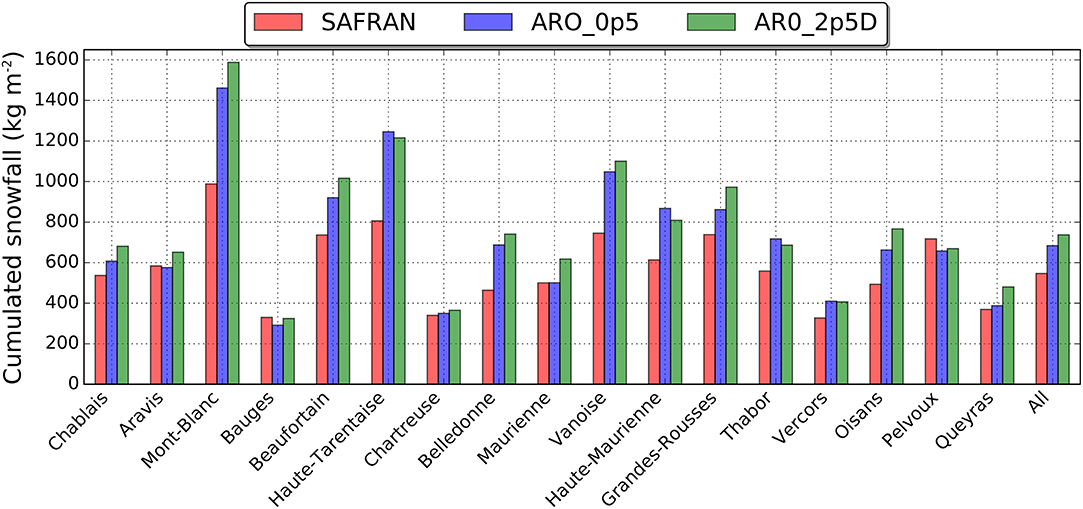
Figure 3. Total snowfall from September 1st 2011 to June 30th 2012 averaged over the massifs shown on Figure 1 for the three precipitation datasets. All refer to the total snowfall for all the massifs. The massifs are ordered from North to South as on Figure 1.
Figure 3 shows large differences between the precipitation datasets. Indeed, seasonal snowfall averaged over all the massifs reaches 546 mm in SAFRAN, 684 mm in ARO_0p5, and 737 mm in ARO_2p5D. The largest differences (over 30%) between SAFRAN and the two datasets derived from AROME are found in high-altitude massifs located in the inner French Alps (Mont Blanc, Haute Tarentaise, Vanoise, Haute Maurienne, and Oisans; Figure 1). Figures 2E,F show that ARO_2p5D and ARO_0p5 have similar patterns of differences of cumulated snowfall with SAFRAN. ARO_2p5D estimates more snowfall than ARO_0p5 over the massifs, except for three of them located along the border with Italy (Haute Tarentaise, Haute Maurienne, and Thabor).
Four cross sections of total snowfall and topography from north-west to south-east are shown on Figure 4 to illustrate more in details the differences between the datasets. These transects are aligned along the general axis of prevailing westerlies and northwesterlies and cut through the main mountain ranges of our study area. Overall, ARO_0p5 and ARO_2p5D agree well in their estimation of seasonal snowfall across all transects. Local differences can be found near the summits of the different massifs but no systematic pattern can be identified. Figures 4A,C also suggest that ARO_0p5 simulates less snowfall on the leeside of some mountain ranges (Chablais on Figure 4A; Grandes Rousses and Pelvoux on Figure 4C). When compared to SAFRAN, a good agreement is found for regions of low elevation (below 1,500 m; Bauges on Figure 4B; Chartreuse on Figure 4C). At higher elevation, the estimations of ARO_0p5 and ARO_2p5D diverges from the one provided by SAFRAN. The largest discrepancies between the datasets are found at the first significant topographic barriers on the windward side of the different mountain ranges. For example, the 2,000-m topographic rise at the border between the Maurienne and the Vanoise massifs leads to snow accumulation for the two datasets based on AROME that can reach three times the value estimated with SAFRAN (Figure 4B). Similar effects are found for the Mont Blanc massif (Figure 4A) and the Belledonne and Grandes Rousses ranges (Figure 4C). Regions of lower estimations of seasonal snowfall in ARO_0p5 and ARO_2p5D compared to SAFRAN are generally found on the leeward side of the main mountain ranges (Pelvoux on Figure 4C; Vercors on Figure 4D). SAFRAN does not present the variability between the upwind and downwind sides of the massifs because of its assumption of climatological homogeneity (section 2.2.2).
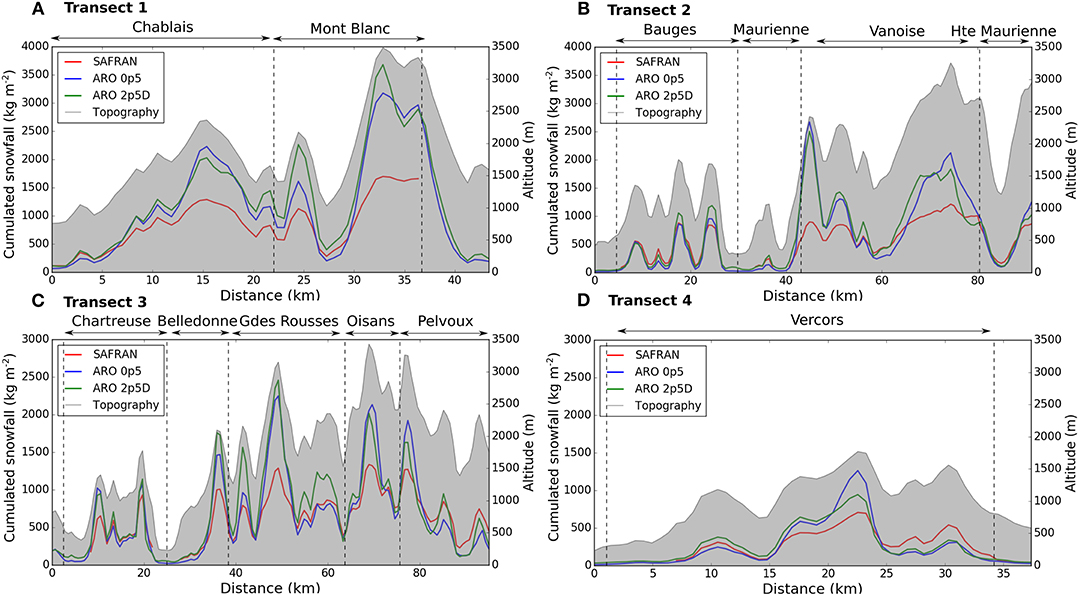
Figure 4. Cross sections of total snowfall from September 1st 2011 to June 30th 2012 for AROME 500 m (blue), downscaled AROME 2.5 km (green), and SAFRAN (red) along four transects, with topography plotted on the right axis in gray. The location of the transects is given in Figure 2. The name of the SAFRAN massifs crossed by the transect is given above each graph. Note that the transect length varies from one figure to another.
3.2. Snowpack Simulations
The three precipitation datasets described in the previous section were used in combination with other atmospheric forcing from AROME 500 m to drive snowpack simulations with Crocus. These simulations are evaluated below in terms of snow depth, snow-covered area and snow accumulation over glaciers.
3.2.1. Snow Depth
Table 2 provides detailed error statistics for simulated snow depth for different time periods throughout the winter and several elevation bands. When all elevations are considered, the best performances (lower RMSE and bias) are obtained with SAFRAN for the whole snow season as well as for the different sub-periods. ARO_0p5 brings improvements when compared to ARO_2p5D with a reduction of 9% in the overall RMSE and 33% in the overall bias. However, both simulations still present large positive biases and large RMSE that are mainly explained by model performances above 1,500 m. Indeed, below 1,500 m, the three snowpack simulations give similar results with a slight tendency to underestimate snow depth, especially for ARO_0p5 and SAFRAN in mid-winter (January to March). On the contrary, above 1500 m, positive biases and large RMSE are found with ARO_0p5 and ARO_2p5D. They increase with elevation and are maximal above 2,000 m in late snow season (April to June).
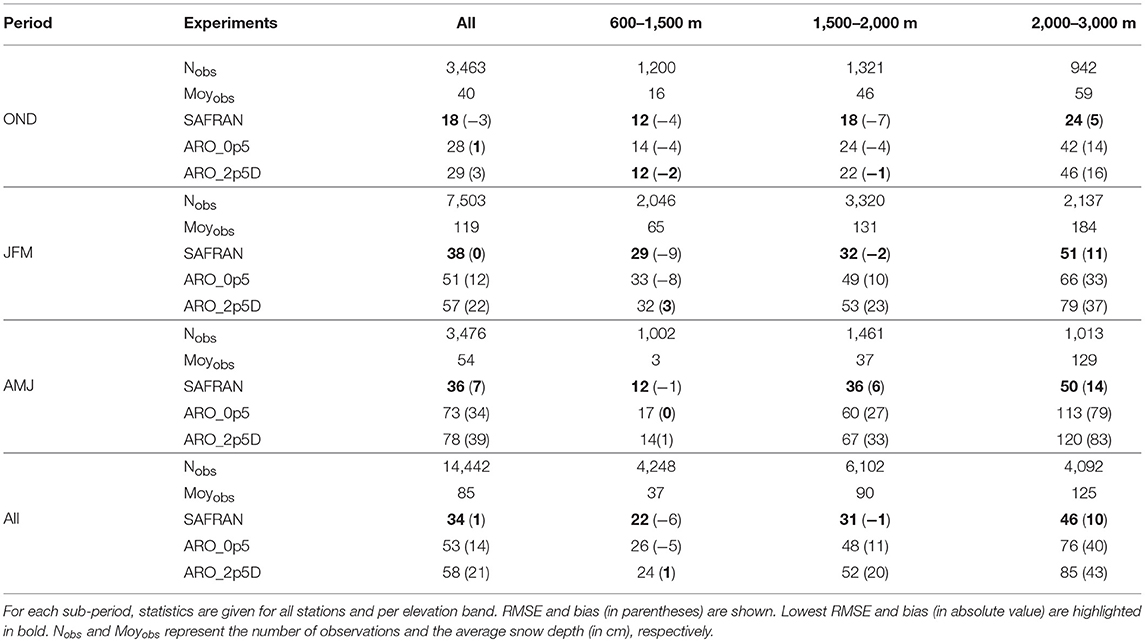
Table 2. Error statistics (in cm) for simulated snow depth against observations for winter 2011-2012 for four time periods: (1) October–December (OND), (2) January–March (JFM), (3) April–June (AMJ), and (4) over the entire snow season (All).
We then used snow depth time series to study more in details positive daily snow depth variations, ΔHS, and how they were related to the errors statistics in snow depth presented in Table 2. Figures 5, 6 show the ETS and the total amount of observed and simulated ΔHS for different categories. The same elevation bands as in Table 2 were considered. For all precipitation datasets and elevation bands, the skill decreases with increasing accumulation threshold. SAFRAN presents the best skill for thresholds up to 10 cm per 24 h for all the elevation bands (Figure 5). ARO_0p5 and ARO_2p5D have similar skills for thresholds up to 10 cm per 24 h whereas ARO_2p5D shows the best skill for the 20 cm per 24 h threshold. For higher thresholds, the limited sample sizes make impossible any reliable interpretation.
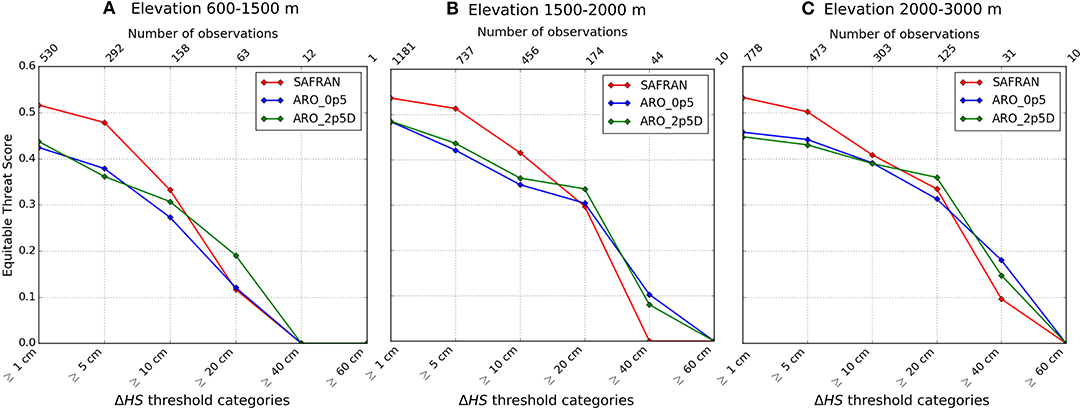
Figure 5. Equitable Threat Score (ETS) for daily snow depth variations, ΔHS, for different thresholds for the different snowpack simulations and for several elevation bands: (A) 600–1,500 m, (B) 1,500–2,000 m, and (C) 2,000–3,000 m. The upper x axis shows the number of observations per category.
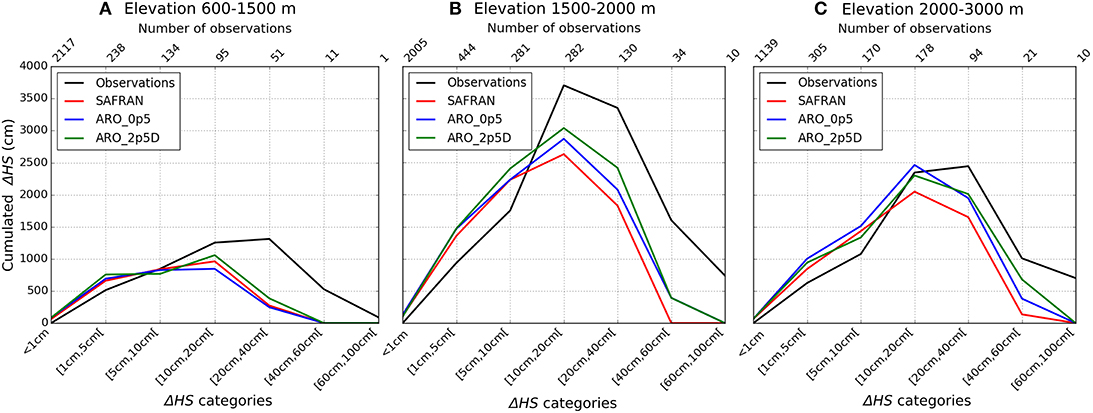
Figure 6. Cumulated daily snow depth variations, ΔHS, by categories in the observation dataset and the different snowpack simulations for several elevation bands: (A) 600–1,500 m, (B) 1,500–2,000 m, and (C) 2,000–3,000 m. The upper x axis shows the number of observations per category.
The total amount of snow accumulation in the observations and the simulations is larger between 1,500 and 2,000 m (Figure 6B) since more observations are available for this elevation band (see section 2.4.1 and the upper x axis of each graph on Figure 6). For all elevation bands, the lowest snow accumulation category (1–5 cm) is overestimated for all Crocus simulations. It is also the case for the category (5–10 cm) above 1,500 m. On the contrary, higher snow accumulation categories are underestimated by all Crocus simulations. Below 1500 m, the three precipitation datasets lead to similar results for higher categories: daily snow accumulation in the range 20–40 cm is strongly underestimated while no daily accumulation above 40 cm is captured. Above 1,500 m, the underestimation is more pronounced by SAFRAN than by ARO_0p5 and ARO_2p5D in the range 20–60 cm. In this range, ARO_2p5D is systematically closer to the observations than ARO_0p5. Finally, no daily accumulation larger than 60 cm is captured by the simulations above 1,500 m.
Positive daily snow depth variations were then cumulated for 26 stations covering the different elevation bands. Figure 7 shows the example of time series of snow depth and cumulative sum of positive daily snow depth variations, ΣΔHS+, for two stations located above 2,000 m. Figure 8 compares observed and simulated ΣΔHS+ at the end of the winter and shows an overall increase in observed ΣΔHS+ with elevation. Observed ΣΔHS+ larger than 600 cm at the end of the winter are found for five stations, all located above 2,000 m. The general underestimation of ΔHS above 10 cm (Figure 6) leads to an underestimation of ΣΔHS+ at the end of the winter for all simulations. It reaches -31 % for SAFRAN (Figure 8A), -22 % for ARO_0p5 (Figure 8B) and -18 % for ARO_2p5D (Figure 8C). SAFRAN has the highest coefficient of determination despite its overall negative bias. Among the two datasets based on AROME, ARO_0p5 has the best performances with a higher coefficient of determination and a lower RMSE than ARO_2p5D.
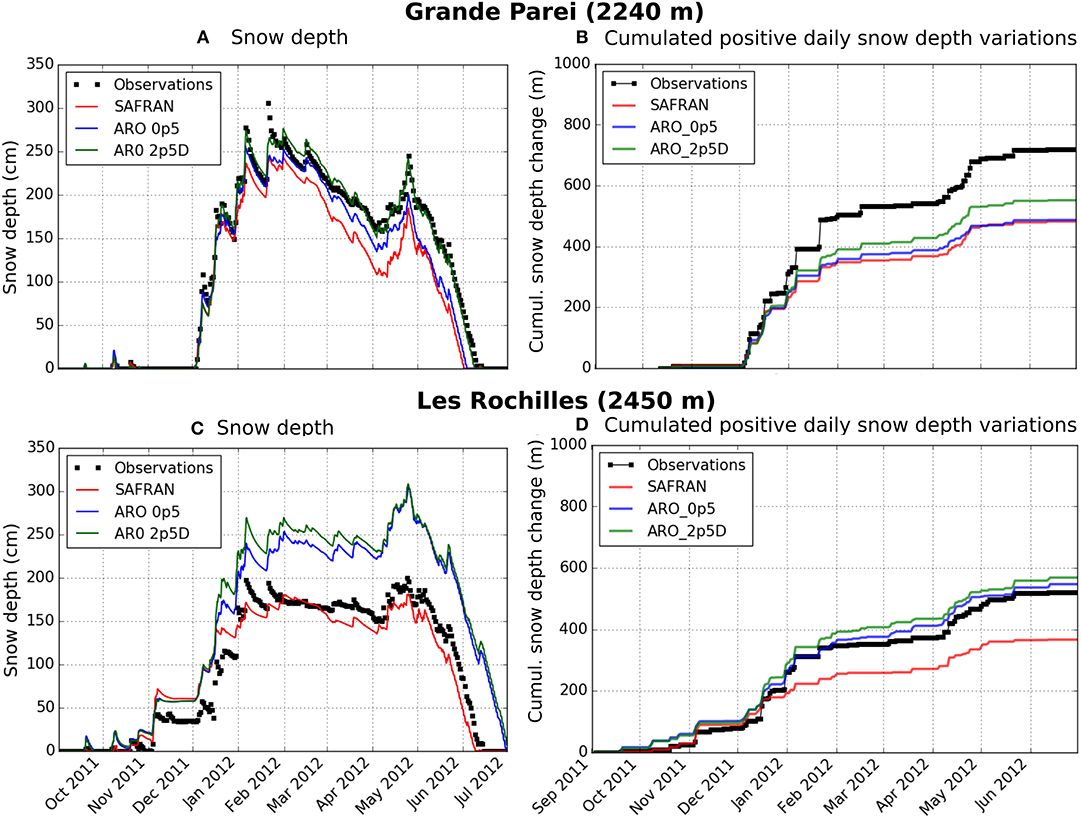
Figure 7. Times series of simulated and observed snow depth and cumulative sum of positive daily snow depth variations at 2 stations: (A,B) Grande Parei and (C,D) Les Rochilles. The location of the stations is shown on Figure 1.
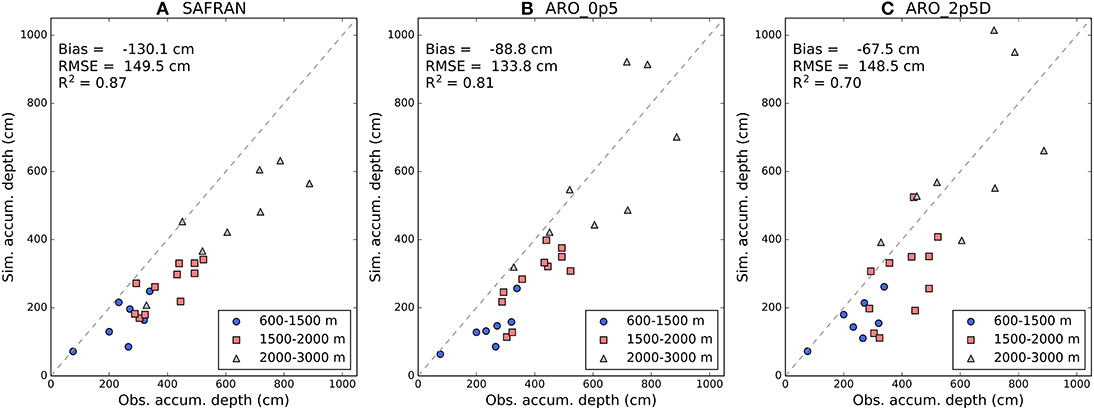
Figure 8. Comparison of measured and simulated cumulative sum of positive daily snow depth variation at the end of the season for 26 stations. Results of three Crocus simulations are considered: (A) SAFRAN, (B) ARO_0p5, and (C) ARO_2p5D. On each graph, the dashed line represents the line y = x and different symbols are used as a function of the stations elevation. Error statistics (Bias, RMSE, and coefficient of determination, R2) are given for each simulation.
Figure 9 compares the biases in simulated snow depth computed from October 1st to June 30th for 26 stations with the biases in simulated cumulative sum of positive daily snow depth change, ΣΔHS+, computed over the same period. It shows that a positive bias in ΣΔHS+ systematically led to a positive bias in simulated snow depth (see for example Les Rochilles station on Figures 7C,D). This is especially the case for ARO_2p5D (Figure 9C) for which 7 among 8 snow depth biases above +50 cm were associated with positive biases in ΣΔHS+. On the contrary, no clear relationship can be identified for stations presenting a negative bias in ΣΔHS+. For SAFRAN (Figure 9A), 15 stations have a positive bias in snow depth among the 25 ones with a negative bias in ΣΔHS+. Similar conclusions are reached for ARO_0p5 (Figure 9B) and ARO_2p5D (Figure 9C). This shows that biases in positive daily snow depth change are not the only processes responsible for the generation of biases in simulated snow depth as already reported in Quéno et al. (2016) and Luijting et al. (2018). For example, the symbols highlighted in red on Figure 9 correspond to a high-altitude station located in the Oisans massif (Figure 1). A detailed analysis of the snow depth time series at this station (not shown) revealed that this station was exposed to wind-induced snow erosion which is not simulated by the model. Therefore, biases in cumulated positive daily snow depth variations do not translate necessarily directly into biases in snow depth.
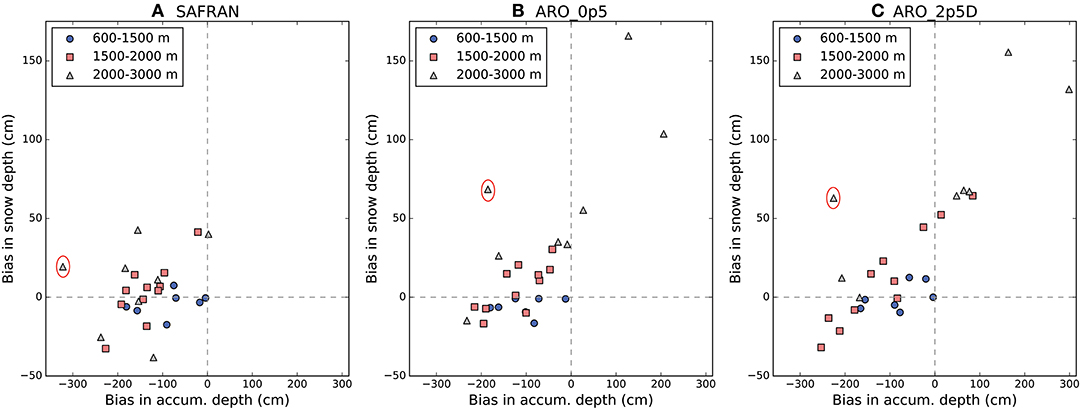
Figure 9. Comparison of snow depth biases computed from October 1 to June 30 with biases in cumulative sum of positive daily snow depth change over the same period at 26 stations. Results of three Crocus simulations are considered: (A) SAFRAN, (B) ARO_0p5, and (C) ARO_2p5D. On each graph, different symbols are used as a function of the stations elevation. The red circles highlight the results at a specific station which are discussed in the text.
3.2.2. Snow Cover Area
Figure 10 compares simulated and observed snow covered area at three stages of the snow season: (i) beginning of winter (November 11th 2011), (ii) end of winter (March 16th 2012) and (iii) mid-Spring (May 14th 2012). On November 11th, no snow is present on the foothills of the French Alps in the observation as well as in the different simulations (except at high elevation for the Chablais and Aravis massifs). For the inner massifs, ARO_0p5 and ARO_2p5D tend to overestimate the snow cover compared to SAFRAN and MODIS in several areas characterized by a strong topographic enhancement of snowfall in AROME and identified on Figure 4. This is for example the case of the peaks lying at the northwestern side of the Vanoise massif. On March 16th, snow cover is present for all the massifs for the three simulations. Only the large valleys of the French Alps are snow free. This pattern is consistent with the MODIS data but the snow cover area is less extended in the observations for the massifs of the foothills (Vercors, Chartreuse, Bauges). These massifs are partially covered by forests (see Supplement Section 2) which (i) alter the quality of the MODIS snow product in these regions (e.g., Parajka et al., 2012) and (ii) make snowpack simulations less reliable in these regions since the model was not configured to account for snow/canopy interactions. On May 14th, ARO_0p5, ARO_2p5D, and SAFRAN in a lower extent show a large overestimation of snow cover on the foothills and a better agreement for the inner massifs.
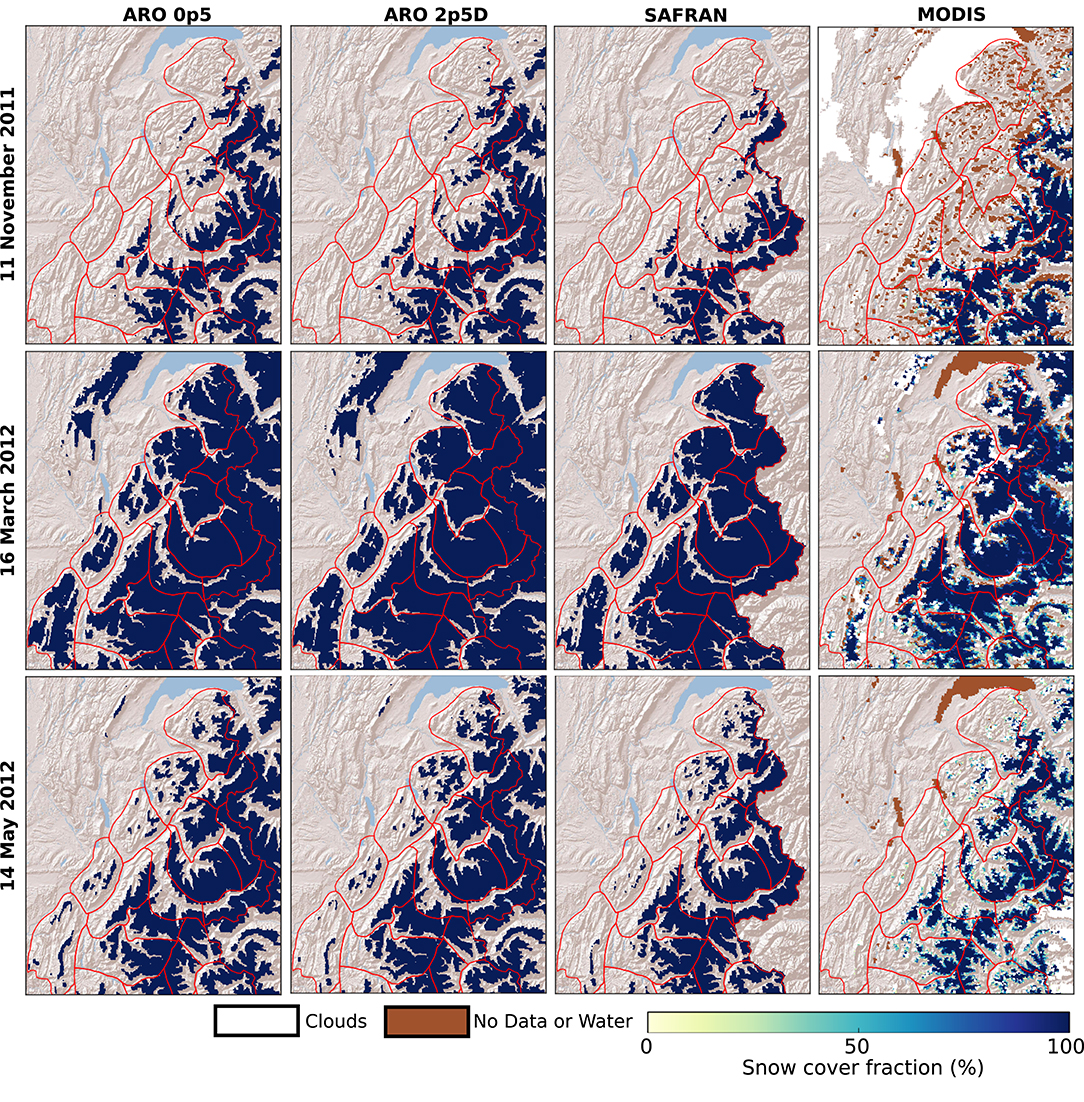
Figure 10. Maps of snow covered fractions simulated by Crocus driven by the three forcing datasets and observed by MODIS (Right) for three dates of winter 2011–2012: 11 November 2011 (Top), 16 March 2012 (Middle) and 14 May 2012 (Bottom). Results of SAFRAN experiment are only shown within the limit of the massifs (red lines).
A more quantitative comparison of model performances is given on Figure 11. It shows the temporal evolution of snow cover area and Jaccard Index for different elevation bands. The evaluation is only carried out for non-forested areas as mentioned in section 2.4.2. For November 2011, the simulated SCA remains nearly constant in all three Crocus simulations and tends to be higher than MODIS SCA, especially above 2500 m. In this elevation band, MODIS SCA slightly decreases in November, partially due to an increase in the number of missing data due to low incidence on north-facing slopes. SAFRAN better captures than ARO_0p5 and ARO_2p5D the spatial variability of the snow cover during this period (higher Jaccard Index). The large increase in SCA in December is then well-captured by the different Crocus simulations at all elevations and followed by a mid-winter period (January and February) characterized by a full snow coverage and Jaccard index near 1 above 1,500 m in all simulations. Below this elevation, all simulations overestimate SCA in mid-winter with ARO_0p5 performing best (lowest differences with observation). However, it shows similar performances in terms of snow cover similarity compared to SAFRAN and ARO_2p5D. From March 2012, all Crocus simulations tend to overestimate the snow cover area during the melting period, especially for ARO_0p5 and ARO_2p5D which is consistent with the overestimation of snow depth reported in Table 2 for the period April-June. SAFRAN provides the best agreement in terms of snow cover similarity during this period.
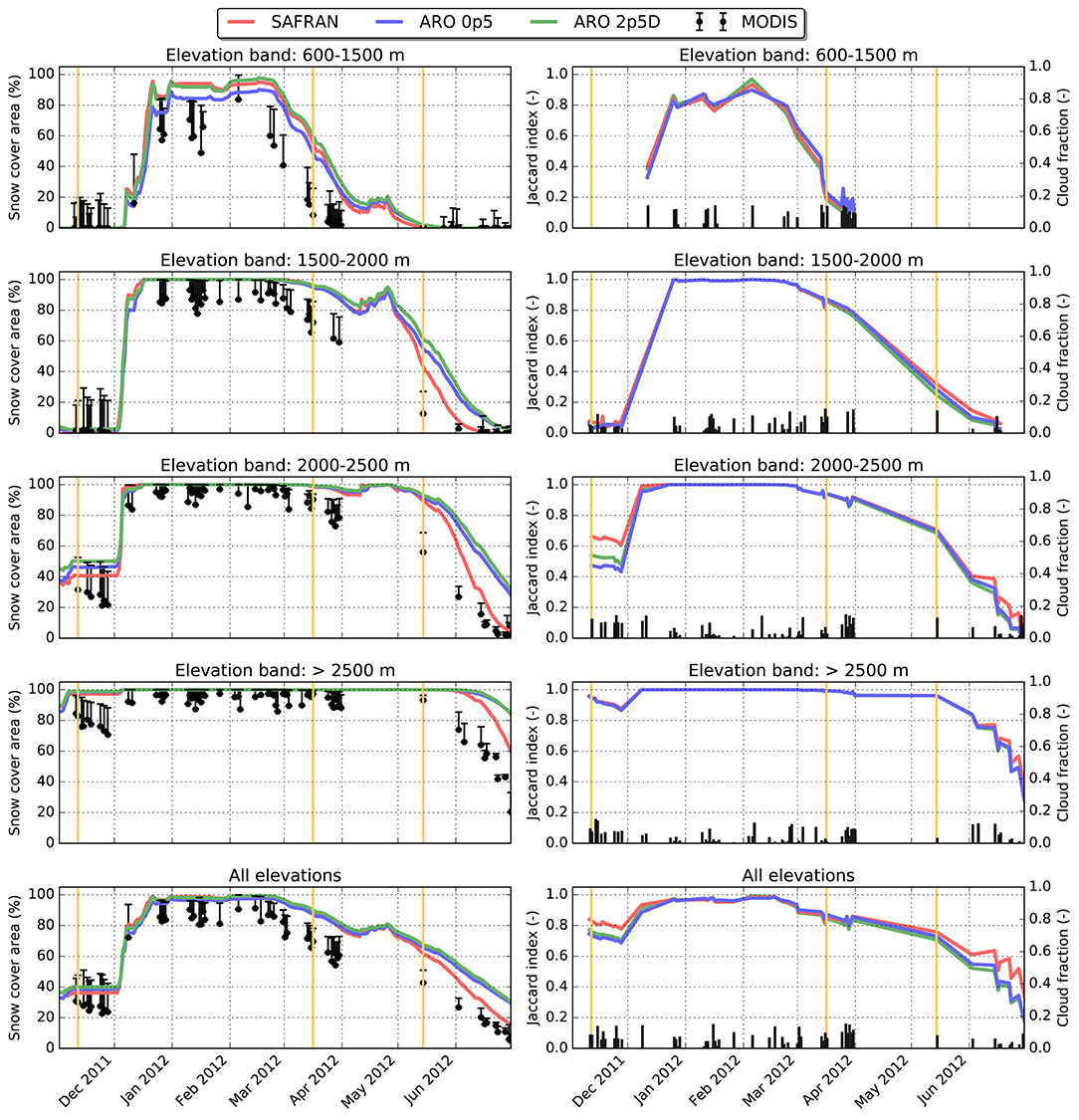
Figure 11. (Left) Temporal evolution of observed and simulated snow cover area for several elevation bands and for all elevations. Vertical error bars for MODIS observations show the uncertainty associated with cloud presence and missing data; (Right) Temporal evolution of Jaccard index for the three Crocus experiments. The vertical bars represent the cloud coverage. Only MODIS images with a cloud cover below 15% were used to compute the Jaccard index. The vertical orange lines on each graph represent the dates shown on Figure 10.
3.2.3. Glacier Winter Mass Balance
We used winter surface mass balance (WSMB) measurements at point locations of six glaciers (Table 1 and Figure 1) to provide complementary evaluation data, in particular at high elevation. Indeed, above 2,500 m, only five stations measuring snow depth were used in section 3.2.1 and MODIS data bring only limited information since snow was present most of the time between November and June at high elevation (Figure 10). Table 3 provides the overall error statistics of simulated WSMB for each glacier while Figure 12 shows the observed and simulated WSMB as a function of elevation for each glacier. A detailed analysis (not shown) revealed that the average contribution of ablation (surface sublimation and melt) on simulated WSMB is just 1.1% with a maximal contribution of 6.3% for the Crocus simulation driven by SAFRAN precipitation for the lowest drilling cores on the Argentière glacier. Therefore, differences in WSMB between the Crocus simulations are mainly explained by differences in the precipitation forcing.
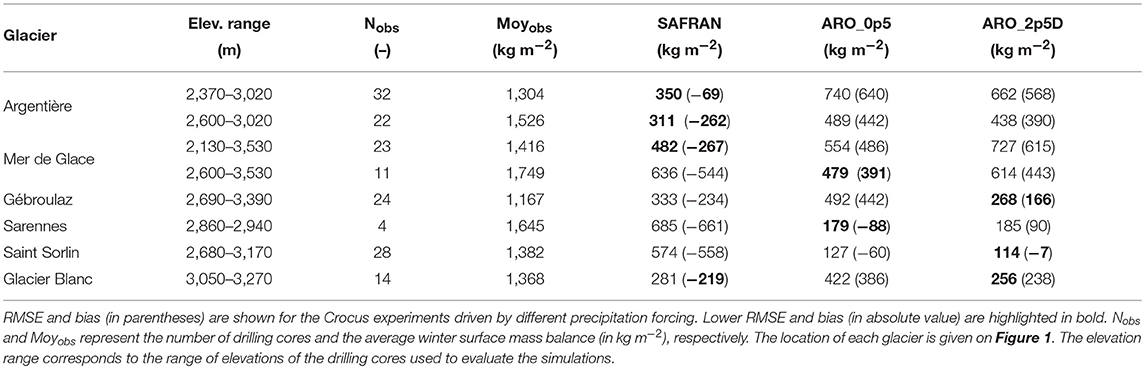
Table 3. Error statistics (in kg m−2) for simulated winter surface mass balance against observations collected at drilling cores for six glaciers of the French Alps for winter 2011–2012.
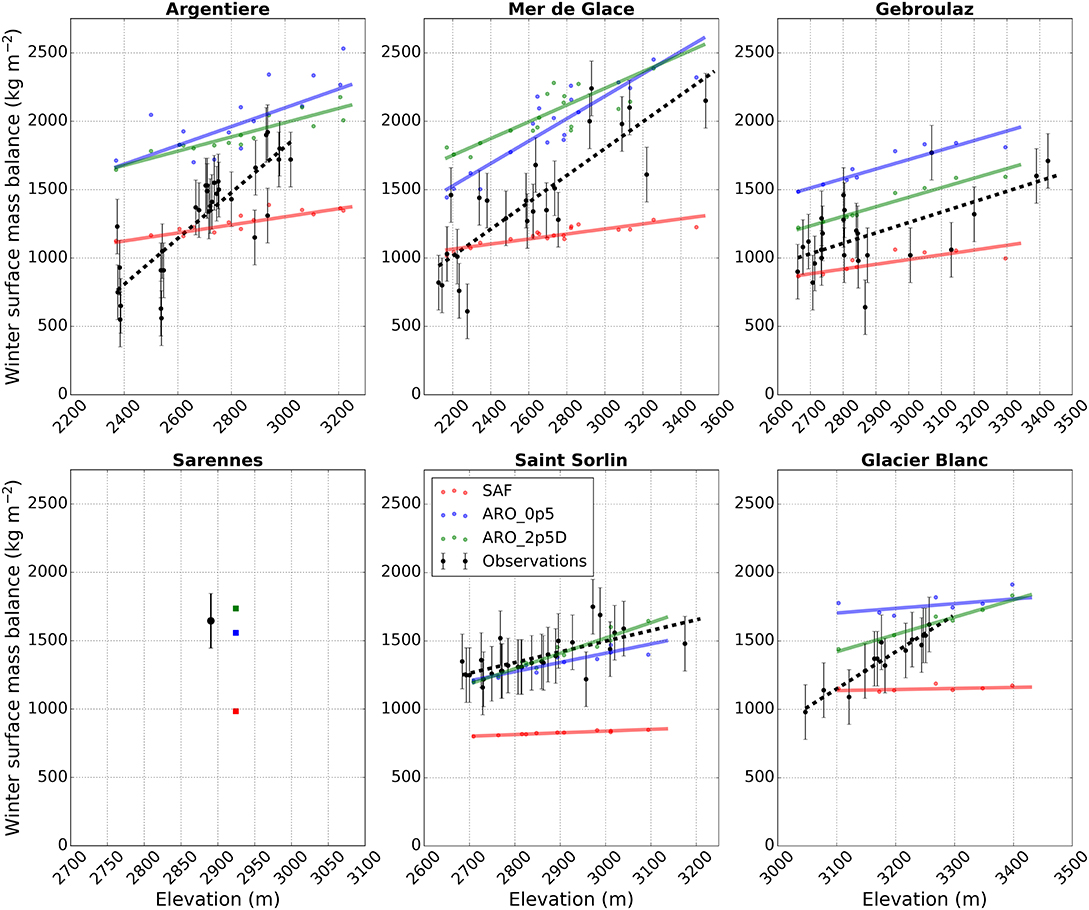
Figure 12. Winter surface mass balance (in kg m−2) for winter 2011–2012 of six glaciers of the French Alps as a function of elevation derived from observations and simulated with Crocus driven by the three forcing datasets. The location of each glacier is given on Figure 1. For each dataset, the line represents the best-fitted linear regression of winter surface mass balance as a function of elevation. No regression line is shown for Sarennes glacier since it covers a too narrow elevation band. The error bars on the observation points represent the typical measurement error. Note that different elevation ranges are used for each glacier.
Among these glaciers, two of them are located in the Mont Blanc massif and cover large elevation ranges: the Argentière and Mer de Glace glaciers (Figure 1). The largest differences in seasonal snowfall between SAFRAN and the two versions of AROME were found in this region (Figures 2, 3). In terms of WSMB, SAFRAN experiment provided the best results (lower RMSE and bias) for both glaciers. However, it did not capture the increase in snow accumulation with elevation and strongly underestimated WSMB above 2,600 m (Table 3). Both simulations driven by AROME led to large overestimated WSMB (Figure 12). Above 2,600 m, this overestimation ranges between 22 and 29% depending on the glacier and on the version of AROME. Figure 12 also shows that the three Crocus simulations cannot reproduce the spatial variability of snow accumulation over these two glaciers which is strongly influenced by local processes, such as preferential deposition, wind-driven snow transport as well as gravitational snow transport (Réveillet et al., 2017) that are not implemented in the model. This point is further discussed in section 4.
The other four glaciers are all located above 2,600 m. SAFRAN experiment systematically underestimated WSMB of each of these glaciers, especially for the Sarennes and Saint Sorlin glaciers (Grandes Rousses massif, Figure 1) where the underestimation reaches −40%. For these two glaciers, ARO_0p5 and ARO_2p5D provide estimations of WSMB within the range of uncertainty of the measurements. This suggests that ARO_0p5 and ARO_2p5D capture well high-elevation snowfall for this massif but without fully reproducing the spatial variability of snow accumulation for Saint Sorlin glacier. Finally, for the Gébroulaz glacier and the Glacier Blanc, ARO_0p5 overestimates the winter snow accumulation by 38 and 28%, respectively. Improved performances are obtained with ARO_2p5D with an overestimation decreasing to 14% for Gébroulaz glacier and 18% for Glacier Blanc.
4. Discussion
4.1. Precipitation Datasets in the French Alps
This paper first compared three precipitation datasets at 500-m grid spacing in the French Alps: two of them were derived from the NWP system AROME (ARO_0p5: dynamical downscaling from AROME 2.5 km; ARO_2p5D: simple downscaling from AROME 2.5 km) and the third one was obtained from the SAFRAN analysis. The two precipitation datasets based on AROME show larger areal-mean total snowfall than SAFRAN for most of the massifs considered in this study (Figure 3). Large differences between AROME and SAFRAN are found in (i) areas of high-elevation and (ii) along the topographic barriers located on the windward side of the different mountain ranges (Figure 4). These differences are consistent with the results of Vionnet et al. (2016) obtained for AROME 2.5 km. At high elevation, the differences between AROME and SAFRAN are similar to the differences obtained between the WRF atmospheric model and the gauge-based PRISM dataset for different mountains ranges of the US (e.g., Gutmann et al., 2012; Silverman et al., 2013; Jing et al., 2017). They are partially explained by the limited number and reliability of stations measuring precipitation at high-elevation used in SAFRAN in the French Alps or PRISM in the US. In addition, the climatological vertical profile used as the initial precipitation guess in SAFRAN presents uncertainties at high-elevation. The topographic barriers along on the windward side of the mountain ranges correspond to areas of strong vertical motions in AROME which, combined with moisture supply, favor large condensation rates and enhanced snowfall, similar to what was found by Rasmussen et al. (2011) with WRF in the mountains of Colorado. SAFRAN cannot reproduce this intra-massif variability of snowfall, due to its assumption of climatological homogeneity within each massif.
The two precipitation datasets based on AROME led to similar spatial patterns of seasonal snowfall with snowfall contours that closely follow topography contours (Figure 2). This illustrates the strong influence of the underlying topography on the precipitation simulated by a high-resolution NWP system (Rasmussen et al., 2011; Hughes et al., 2017; Jing et al., 2017). On average over the French Alps, AROME 500 m simulated 7% less total snowfall than AROME 2.5 km with simple downscaling (Figure 3). This differs from the work of Jing et al. (2017) that obtained a slight increase (+1.3%) in mean snowfall with WRF at 1.3 km compared to WRF at 4 km in the Yellowstone area in the US. Differences between ARO_0p5 and ARO_2p5D can be explained by (i) a finer representation of terrain-induced precipitation in ARO_0p5 and (ii) the value of the precipitation-elevation adjustment factor used to downscale the precipitation of AROME 2.5 km. Indeed, this factor has been derived by Thornton et al. (1997) using measurements from rain gauges in the Northwestern USA. It is widely used for distributed snowpack modeling (Liston and Elder, 2006). This factor could be adjusted for the French Alps using precipitation dataset specific of this area (Gottardi et al., 2012).
4.2. Impact on Snowpack Simulations
Snowpack simulations driven by ARO_0p5 and ARO_2p5D led to a general overestimation of snow depth above 1,500 m that increases with elevation (Table 2). Using SAFRAN precipitation reduced the positive bias in snow depth above 1,500 m. Quéno et al. (2016), Vionnet et al. (2016), and Luijting et al. (2018) reached similar conclusions in terms of total snow depth using AROME at 2.5 km to drive Crocus in the Pyrenees, the French Alps and Norwegian mountains. Errors statistics in snow depth are nonetheless improved for ARO_0p5 compared to ARO_2p5D. Quéno et al. (2016) also showed that AROME improved the representation of the snow cover variability compared to SAFRAN in the Pyrenees. Such improvement is not found in our study with SAFRAN showing a better representation of snow-covered area observed by MODIS than ARO_0p5 and ARO_2p5D in fall and spring time (Figure 11). The overestimation of snow accumulation in regions of orographic precipitation enhancement simulated by AROME may explain the differences during the fall (Figure 10). In springtime, the overestimation of snow cover can be associated with the general overestimation of snow depth above 1,500 m in ARO_0p5 and ARO_2p5D.
Daily snow depth variations were considered to analyze more in details the results obtained with the different precipitation datasets as in Quéno et al. (2016) and Currier et al. (2017). Figure 6 shows that all precipitation datasets led to an underestimation of daily snow depth change larger than 10 cm that resulted in an underestimation of cumulated daily snow depth increase with ARO_0p5 providing the best performances (lowest RMSE) among the three datasets (Figure 8). Recently, Champavier et al. (2018) found the same underestimation for SAFRAN-Crocus at 10 stations measuring daily new snow depth on snow boards in the French Alps. The general overestimation of snow depth with AROME is not explained by an overestimation of snowfall height (except at some stations) as shown on Figure 9, confirming the earlier findings of Quéno et al. (2016). In particular, as in Quéno et al. (2016), wind-induced snow erosion, not simulated in the configuration of Crocus used in our study, can explain part of the overall positive biases in snow depth found for high-altitude stations. In addition, errors in daily snow depth change are not only explained by errors in daily precipitation amount and phase. Indeed, they are affected by errors in simulated new snow density and new snow compaction in Crocus. Helfricht et al. (2018) have shown that the parameterization of Crocus consistently overestimated new snow density at four well-instrumented sites in the European Alps. This can explain the consistent underestimation of daily snow depth increase found with our three precipitation datasets. On the other hand, Quéno et al. (2016) found an underestimation of snow compaction in Crocus that can compensate for the underestimation of daily snow depth increase when considering error statistics for total snow depth. This illustrates the difficulty of evaluating precipitation datasets on snow height because this variable involves the modeling of other uncertain processes (in particular, density of falling snow, snow compaction) and might not be representative of errors in snow mass in some cases.
Measurements of winter surface mass balance for 6 glaciers were considered to provide additional evaluation data, especially at high-elevation where few stations measuring snow depth are available. Our study showed a systematic underestimation of high-altitude snow accumulation over the six glaciers using SAFRAN precipitation (Figure 12). This underestimation increases with elevation. These results are in agreement with previous studies (Gerbaux et al., 2005; Réveillet et al., 2017, 2018; Revuelto et al., 2018). Using 17 years of measurements, Réveillet et al. (2017) found that SAFRAN solid precipitation are underestimated by a factor 1.5 on average at the glacier scale for the Argentière, Mer de Glace, Saint Sorlin and Gébroulaz glaciers. In our study, the average factor reaches 1.3 for these four glaciers for winter 2011/2012 (Table 3), in the range of inter-annual variability reported by Réveillet et al. (2017). Compared to SAFRAN, ARO_0p5 and ARO_2p5D brought substantial improvements for the Sarennes and Saint Sorlin glaciers located in the Grandes Rousses ranges (Figure 12). For the rest of the glaciers, they led to an overestimation of WSMB, especially for the Argentière and Mer de Glace glaciers in the Mont Blanc massif where ARO_0p5 and ARO_2p5D simulate the largest seasonal snowfall in the French Alps (Figure 3). This illustrates that the quality of AROME precipitation at high-altitude is highly variable across the different massifs of the French Alps. Despite the overestimation of WSMB, ARO_0p5 and ARO_2p5D capture well the relative change of WSMB with elevation for the Mer de Glace and Gébroulaz glaciers, suggesting that these systems can provide relevant information on the local gradient of precipitation with elevation. Further specific work is required to better understand the relative contribution of snowfall variability with elevation and local processes (preferential deposition, wind-induced and gravitational snow transport) in determining the final pattern of snow accumulation over each of these glaciers. The evaluation of snow depth simulations was restricted to 87 stations and the WSMB of glaciers was evaluated at 125 drilling cores. As mentioned in sections 2.4.1, 2.4.3, these point measurements are affected by a limited spatial representativity and coverage. High-resolution maps of snow depth from airborne platforms (LiDAR or photogrammetry) (e.g., Helfricht et al., 2012; Painter et al., 2016; Voegeli et al., 2016) would allow a stronger assessment of model performances over large areas. For example, mountain-range scale snow-cover variability resulting from orographic precipitation (Houze, 2012) is only partially captured by the point measurements. This limits our ability to quantify the expected large impact of the assumption of climatological homogeneity in SAFRAN on the snow cover variability within each massif. Regions of overestimated snow depth due to the strong orographic precipitation enhancement in AROME would be also more clearly identified. In addition, snow depth measurements from airborne platforms would allow to quantify the impact of small-scale winter precipitation processes (cloud-dynamical processes and advection of solid hydrometeors, Gerber et al., 2019) that are not represented in the precipitation datasets used in our study. Over glaciers, the quantification of the snow accumulation from LiDAR or photogrammetry requires to take into account the effects of the glacier dynamics (Sold et al., 2013). Finally, the comparison between simulated and observed high-resolution maps of snow depth would benefit from the explicit simulation of wind-induced snow transport (e.g., Vionnet et al., 2014) and gravitational snow transport (Bernhardt et al., 2010) in our distributed snowpack simulations.
In our study, the influence of the different precipitation datasets on snowpack simulations were quantified and discussed in simulations where all the atmospheric driving data, except the precipitation, were obtained from AROME 500 m. These forcing were potentially affected by biases that impact the snowpack simulations (Raleigh et al., 2015). In particular, errors in the longwave and shortwave radiative forcing represent a large source of uncertainty (Quéno et al., 2018) and impact the simulated energy balance and resulting snow melt. Errors in wind speed can also affect the magnitude of turbulent fluxes simulated by Crocus and the resulting melt (Réveillet et al., 2018). They will also affect the density of falling snow (Vionnet et al., 2012) leading to potential errors in simulated daily snow accumulation. Snowpack simulations driven by an ensemble of atmospheric forcing (Vernay et al., 2015) could be used to better estimate these uncertainties. Simulated total snow depth is also affected by the uncertainty on various processes in the snowpack model especially those affecting the surface energy balance, in particular the turbulent fluxes (Schlögl et al., 2016). It is expected to be lower than forcing uncertainty (Raleigh et al., 2015) but can still have a significant impact on the scores (Essery et al., 2013; Lafaysse et al., 2017).
5. Conclusions
This study aims at evaluating the impact of different precipitation datasets on sub-kilometer snowpack simulations in the French Alps. Toward this goal, we used the NWP system AROME at 500-m grid spacing to produce the atmospheric forcing required to drive the detailed snowpack model Crocus. To assess the impact of the precipitation forcing, we carried out two additional snowpack simulations using precipitation forcing from (i) a simple downscaling of AROME 2.5 km using a precipitation-elevation adjustment factor and (ii) the SAFRAN reanalysis, specially developed for alpine terrain, interpolated at 500-m grid spacing. For these simulations, the rest of the atmospheric driving data were obtained from AROME 500 m. The three snowpack simulations were evaluated against an ensemble of snow observations: (i) ground-based measurements of snow depth at 87 stations, (ii) satellite-derived maps of snow-covered areas and (iii) observations of glacier winter surface mass balance (WSMB) for 6 glaciers of the region. Major conclusions of the model evaluation are summarized below.
• AROME 500 m and AROME 2.5 km with simple downscaling provided similar snowfall patterns strongly influenced by the topography of the French Alps. They significantly differ from the SAFRAN precipitation in (i) areas of high elevation where SAFRAN is less reliable due to the limited number of stations entering the analysis and (ii) along topographic barriers on the windward side of mountain ranges characterized by strong orographic precipitation enhancement in AROME.
• Snowpack simulations driven by the two precipitation datasets obtained with AROME strongly overestimated snow depth above 1,500 m with a positive bias increasing with elevation. It led to an overestimation of snow-covered area in spring time. Crocus driven by SAFRAN precipitation significantly reduced the average positive bias in snow depth and improved the representation of the snow cover evolution.
• Crocus driven by SAFRAN precipitation presented the best skills in estimating daily snow depth increase up to 10 cm per 24 h but strongly underestimated increases above this threshold. AROME 500 m and AROME 2.5 km with simple downscaling improved the estimation of daily new snow depth by Crocus for these categories. Uncertainties in modeling of falling snow density and new snow compaction in Crocus affect these results.
• Cumulated daily snow depth increase over the winter is underestimated by the three snowpack simulations with Crocus driven by SAFRAN precipitation providing the strongest underestimation. It showed that errors in snowfall amount are not the only explanation for the positive biases in total snow depth. Therefore, error statistics in total snow depth have only a limited significance when analyzing the impact of different precipitation datasets on snowpack simulations.
• WSMB measurements for six glaciers provided valuable information on the performances of the different precipitation datasets at high-altitude. Crocus driven by SAFRAN systematically underestimated high-elevation snow accumulation for each glacier. Improvements were obtained with AROME precipitation with non-biased WSMB for two glaciers and an overestimation for the remaining four glaciers. All snowpack simulations in our study did not capture the spatial variability of snow accumulation for the different glaciers due to missing processes, such as wind-induced snow transport, preferential deposition of snowfall and gravitational snow transport.
• The evaluation datasets used in this study suffer from a limited spatial representativeness. High-resolution maps of snow depth derived from airborne platforms are required to further quantify in details the errors in simulated snow depth associated with the assumption of climatological homogeneity for each massif made in SAFRAN, the intensity of the orographic precipitation enhancement in AROME and the role of near-surface winter precipitation processes not captured by the three precipitation datasets.
This study constitutes the first evaluation of the potential and limitations of a sub-kilometer NWP system for snowpack and glacier mass balance modeling in alpine terrain. Only little improvement was found compared to the precipitation dataset derived from AROME 2.5 km with a simple downscaling. Further multi-year evaluation is required to better assess the performance of AROME 500 m for contrasted winter seasons. This study placed a specific emphasis on precipitation but sub-kilometer NWP system can also bring valuable information in complex terrain for other meteorological variables, such as wind speed and direction (e.g., Horvath et al., 2012; Vionnet et al., 2015). This can potentially improve the simulation of wind-induced snow accumulation in high-resolution snowpack simulations (e.g., Vionnet et al., 2014).
Data Availability
The datasets generated for this study are available on request to the corresponding author.
Author Contributions
VV and DS designed the study. VV designed the modeling strategy and was responsible for the preparation of the manuscript. LA ran the numerical experiments with AROME 500 m. ID-E contributed to the preparation of the atmospheric forcing. ML provided the snowpack model and the preprocessing toolkit. DS, ET, CV, and MR collected and provided glacier mass balance data. MD provided the MODIS data. LQ made the comparison between snow cover simulated by Crocus and observed by MODIS. All authors contributed to the preparation of the manuscript.
Funding
Institutional funding from CNRM (Météo France/CNRS) is acknowledged.
Conflict of Interest Statement
The authors declare that the research was conducted in the absence of any commercial or financial relationships that could be construed as a potential conflict of interest.
The handling editor declared a past co-authorship with the authors DS and CV.
Acknowledgments
The authors are grateful to B. Vincendon, S. Riette, Y. Seity, and G. Delautier (Météo France/CNRS, CNRM, Toulouse), A. Rabatel (IGE, Grenoble, France) and J. Etchanchu (CESBIO, Toulouse) for help and discussion. Observations on glaciers are conducted in the framework of the French GLACIOCLIM program (https://glacioclim.osug.fr). We would like to thank everyone who helped in collecting data during the glacier field campaigns. CNRM/CEN, Irstea, and IGE are part of LabEx OSUG@2020 (ANR10 LABX56).
Supplementary Material
The Supplementary Material for this article can be found online at: https://www.frontiersin.org/articles/10.3389/feart.2019.00182/full#supplementary-material
References
Adam, J. C., and Lettenmaier, D. P. (2003). Adjustment of global gridded precipitation for systematic bias. J. Geophys. Res. 108:4257. doi: 10.1029/2002JD002499
Ban, N., Schmidli, J., and Schär, C. (2014). Evaluation of the convection-resolving regional climate modeling approach in decade-long simulations. J. Geophys. Res. Atmos. 119, 7889–7907. doi: 10.1002/2014JD021478
Bernhardt, M., Liston, G., Strasser, U., Zängl, G., and Schulz, K. (2010). High resolution modelling of snow transport in complex terrain using downscaled MM5 wind fields. Cryosphere 4, 99–113. doi: 10.5194/tc-4-99-2010
Bonekamp, P., Collier, E., and Immerzeel, W. (2018). The impact of spatial resolution, land use, and spinup time on resolving spatial precipitation patterns in the Himalayas. J. Hydrometeorol. 19, 1565–1581. doi: 10.1175/JHM-D-17-0212.1
Brousseau, P., Bouttier, F., Hello, G., Seity, Y., Fischer, C., Berre, L., et al. (2008). A prototype convective-scale data assimilation system for operation: the Arome-RUC. HIRLAM Techn. Rep. 68, 23–30.
Brousseau, P., Seity, Y., Ricard, D., and Léger, J. (2016). Improvement of the forecast of convective activity from the AROME-France system. Q. J. Roy. Meteor. Soc. 142, 2231–2243. doi: 10.1002/qj.2822
Brun, E., David, P., Sudul, M., and Brunot, G. (1992). A numerical model to simulate snow cover stratigraphy for operational avalanche forecasting. J. Glaciol. 38, 13–22.
Brun, E., Martin, E., Simon, V., Gendre, C., and Coleou, C. (1989). An energy and mass balance model of snow cover suitable for operational avalanche forecasting. J. Glaciol. 35, 333–342.
Champavier, R., Lafaysse, M., Vernay, M., and Coleou, C. (2018). “Comparison of various forecast products of height of new snow in 24 hours of French ski resorts at different lead times,” in Proceedings of International Snow Science Workshop (Innsbruck).
Collier, E., and Immerzeel, W. W. (2015). High-resolution modeling of atmospheric dynamics in the Nepalese Himalaya. J. Geophys. Res. Atmos. 120, 9882–9896. doi: 10.1002/2015JD023266
Currier, W. R., Thorson, T., and Lundquist, J. D. (2017). Independent evaluation of frozen precipitation from WRF and PRISM in the Olympic mountains. J. Hydrometeorol. 18, 2681–2703. doi: 10.1175/JHM-D-17-0026.1
Dadic, R., Mott, R., Lehning, M., and Burlando, P. (2010). Wind influence on snow depth distribution and accumulation over glaciers. J. Geophys. Res. 115:F01012. doi: 10.1029/2009JF001261
Daly, C., Halbleib, M., Smith, J. I., Gibson, W. P., Doggett, M. K., Taylor, G. H., et al. (2008). Physiographically sensitive mapping of climatological temperature and precipitation across the conterminous United States. Int. J. Climatol. 28, 2031–2064. doi: 10.1002/joc.1688
Daly, C., Neilson, R. P., and Phillips, D. L. (1994). A statistical-topographic model for mapping climatological precipitation over mountainous terrain. J. Appl. Meteorol. 33, 140–158.
Decharme, B., Boone, A., Delire, C., and Noilhan, J. (2011). Local evaluation of the Interaction between Soil Biosphere Atmosphere soil multilayer diffusion scheme using four pedotransfer functions. J. Geophys. Res. 116:D20126. doi: 10.1029/2011JD016002
Durand, Y., Brun, E., Mérindol, L., Guyomarc'h, G., Lesaffre, B., and Martin, E. (1993). A meteorological estimation of relevant parameters for snow models. Ann. Glaciol. 18, 65–71.
Durand, Y., Laternser, M., Giraud, G., Etchevers, P., Lesaffre, B., and Mérindol, L. (2009). Reanalysis of 44 yr of climate in the french alps (1958–2002): methodology, model validation, climatology, and trends for air temperature and precipitation. J. Appl. Meteorol. Clim. 48, 429–449. doi: 10.1175/2008JAMC1808.1
Essery, R., Morin, S., Lejeune, Y., and Ménard, C. (2013). A comparison of 1701 snow models using observations from an alpine site. Adv. Water Resour. 55, 131–148. doi: 10.1016/j.advwatres.2012.07.013
Fortin, V., Roy, G., Stadnyk, T., Koenig, K., Gasset, N., and Mahidjiba, A. (2018). Ten years of science based on the Canadian precipitation analysis: a CaPA system overview and literature review. Atmos. Ocean 56, 178–196. doi: 10.1080/07055900.2018.1474728
Gardent, M., Rabatel, A., Dedieu, J.-P., and Deline, P. (2014). Multitemporal glacier inventory of the French Alps from the late 1960s to the late 2000s. Glob. Planet. Change 120, 24–37. doi: 10.1016/j.gloplacha.2014.05.004
Gascoin, S., Lhermitte, S., Kinnard, C., Bortels, K., and Liston, G. E. (2013). Wind effects on snow cover in Pascua-Lama, Dry Andes of Chile. Adv. Water Res. 55, 25–39. doi: 10.1016/j.advwatres.2012.11.013
Gerbaux, M., Genthon, C., Etchevers, P., Vincent, C., and Dedieu, J.-P. (2005). Surface mass balance of glaciers in the French Alps: distributed modeling and sensitivity to climate change. J. Glaciol. 51, 561–572. doi: 10.3189/172756505781829133
Gerber, F., Mott, R., and Lehning, M. (2019). The importance of near-surface winter precipitation processes in complex alpine terrain. J. Hydrometeorol. 20, 177–196. doi: 10.1175/JHM-D-18-0055.1
Gottardi, F., Obled, C., Gailhard, J., and Paquet, E. (2012). Statistical reanalysis of precipitation fields based on ground network data and weather patterns: application over French mountains. J. Hydrol. 432, 154–167. doi: 10.1016/j.jhydrol.2012.02.014
Grünewald, T., and Lehning, M. (2015). Are flat-field snow depth measurements representative? A comparison of selected index sites with areal snow depth measurements at the small catchment scale. Hydrol. Proc. 29, 1717–1728. doi: 10.1002/hyp.10295
Gutmann, E., Rasmussen, R., Liu, C., Ikeda, K., Gochis, D., Clark, M., et al. (2012). A comparison of statistical and dynamical downscaling of winter precipitation over complex terrain. J. Clim. 25, 262–281. doi: 10.1175/2011JCLI4109.1
Hagelin, S., Auger, L., Brovelli, P., and Dupont, O. (2014). Nowcasting with the AROME model: first results from the high-resolution AROME airport. Weather Forecast. 29, 773–787. doi: 10.1175/WAF-D-13-00083.1
Helfricht, K., Hartl, L., Koch, R., Marty, C., and Olefs, M. (2018). Obtaining sub-daily new snow density from automated measurements in high mountain regions. Hydrol. Earth Syst. Sci. 22, 2655–2668. doi: 10.5194/hess-22-2655-2018
Helfricht, K., Lehning, M., Sailer, R., and Kuhn, M. (2015). Local extremes in the lidar-derived snow cover of alpine glaciers. Geogr. Ann. A Phys. Geogr. 97, 721–736. doi: 10.1111/geoa.12111
Helfricht, K., Schöber, J., Seiser, B., Fischer, A., Stötter, J., and Kuhn, M. (2012). Snow accumulation of a high alpine catchment derived from lidar measurements. Adv. Geosci. 32, 31–39. doi: 10.5194/adgeo-32-31-2012
Henn, B., Newman, A. J., Livneh, B., Daly, C., and Lundquist, J. D. (2018). An assessment of differences in gridded precipitation datasets in complex terrain. J. Hydrol. 556, 1205–1219. doi: 10.1016/j.jhydrol.2017.03.008
Horton, S., Schirmer, M., and Jamieson, B. (2015). Meteorological, elevation, and slope effects on surface hoar formation. Cryosphere 9, 1523–1533. doi: 10.5194/tc-9-1523-2015
Horvath, K., Koracin, D., Vellore, R., Jiang, J., and Belu, R. (2012). Sub-kilometer dynamical downscaling of near-surface winds in complex terrain using WRF and MM5 mesoscale models. J. Geophys. Res. 117:D11111. doi: 10.1029/2012JD017432
Houze, R. A. (2012). Orographic effects on precipitating clouds. Rev. Geophys. 50:RG1001. doi: 10.1029/2011RG000365
Hughes, M., Lundquist, J. D., and Henn, B. (2017). Dynamical downscaling improves upon gridded precipitation products in the Sierra Nevada, California. Clim. Dyn. doi: 10.1007/s00382-017-3631-z. [Epub ahead of print].
Ikeda, K., Rasmussen, R., Liu, C., Gochis, D., Yates, D., Chen, F., et al. (2010). Simulation of seasonal snowfall over Colorado. Atmos. Res. 97, 462–477. doi: 10.1016/j.atmosres.2010.04.010
Jing, X., Geerts, B., Wang, Y., and Liu, C. (2017). Evaluating seasonal orographic precipitation in the interior western United States using gauge data, gridded precipitation estimates, and a regional climate simulation. J. Hydrometeorol. 18, 2541–2558. doi: 10.1175/JHM-D-17-0056.1
Kochendorfer, J., Nitu, R., Wolff, M., Mekis, E., Rasmussen, R., Baker, B., et al. (2018). Testing and development of transfer functions for weighing precipitation gauges in WMO-SPICE. Hydrol. Earth Sys. Sci. 22, 1437–1452. doi: 10.5194/hess-22-1437-2018
Lafaysse, M., Cluzet, B., Dumont, M., Lejeune, Y., Vionnet, V., and Morin, S. (2017). A multiphysical ensemble system of numerical snow modelling. Cryosphere 11, 1173–1198. doi: 10.5194/tc-11-1173-2017
Lehning, M., Löwe, H., Ryser, M., and Raderschall, N. (2008). Inhomogeneous precipitation distribution and snow transport in steep terrain. Water Resour. Res. 44:W07404. doi: 10.1029/2007WR006545
Liston, G., and Elder, K. (2006). A meteorological distribution system for high-resolution terrestrial modeling (micromet). J. Hydrometeorol. 7, 217–234. doi: 10.1175/JHM486.1
Luijting, H., Vikhamar-Schuler, D., Aspelien, T., Bakketun, Å., and Homleid, M. (2018). Forcing the SURFEX/Crocus snow model with combined hourly meteorological forecasts and gridded observations in southern Norway. Cryosphere 12, 2123–2145. doi: 10.5194/tc-12-2123-2018
Machguth, H., Eisen, O., Paul, F., and Hoelzle, M. (2006). Strong spatial variability of snow accumulation observed with helicopter-borne GPR on two adjacent Alpine glaciers. Geophys. Res. Lett. 33:L13503. doi: 10.1029/2006GL026576
Mankin, J. S., Viviroli, D., Singh, D., Hoekstra, A. Y., and Diffenbaugh, N. S. (2015). The potential for snow to supply human water demand in the present and future. Environ. Res. Lett. 10:114016. doi: 10.1088/1748-9326/10/11/114016
Masson, T., Dumont, M., Mura, M. D., Sirguey, P., Gascoin, S., Dedieu, J.-P., et al. (2018). An assessment of existing methodologies to retrieve snow cover fraction from MODIS data. Rem. Sens. 10:619. doi: 10.3390/rs10040619
Masson, V., Le Moigne, P., Martin, E., Faroux, S., Alias, A., Alkama, R., et al. (2013). The SURFEXv7. 2 land and ocean surface platform for coupled or offline simulation of earth surface variables and fluxes. Geosci. Model Dev. 6, 929–960. doi: 10.5194/gmd-6-929-2013
Mott, R., Scipion, D., Schneebeli, M., Dawes, N., Berne, A., and Lehning, M. (2014). Orographic effects on snow deposition patterns in mountainous terrain. J. Geophys. Res. Atmos. 119, 1419–1439. doi: 10.1002/2013JD019880
Mott, R., Vionnet, V., and Grünewald, T. (2018). The seasonal snow cover dynamics: review on wind-driven coupling processes. Front. Earth Sci. 6:197. doi: 10.3389/feart.2018.00197
Painter, T. H., Berisford, D. F., Boardman, J. W., Bormann, K. J., Deems, J. S., Gehrke, F., et al. (2016). The airborne snow observatory: fusion of scanning lidar, imaging spectrometer, and physically-based modeling for mapping snow water equivalent and snow albedo. Rem. Sens. Environ. 184, 139–152. doi: 10.1016/j.rse.2016.06.018
Parajka, J., Holko, L., Kostka, Z., and Blöschl, G. (2012). MODIS snow cover mapping accuracy in a small mountain catchment–comparison between open and forest sites. Hydrol. Earth Syst. Sci. 16, 2365–2377. doi: 10.5194/hess-16-2365-2012
Quéno, L., Karbou, F., Vionnet, V., and Dombrowski-Etchevers, I. (2018). Satellite-derived products of solar and longwave irradiances used for snowpack modelling in mountainous terrain. Hydrol. Earth Syst. Sci. Discuss. doi: 10.5194/hess-2017-563
Quéno, L., Vionnet, V., Dombrowski-Etchevers, I., Lafaysse, M., Dumont, M., and Karbou, F. (2016). Snowpack modelling in the Pyrenees driven by kilometric-resolution meteorological forecasts. Cryosphere 10, 1571–1589. doi: 10.5194/tc-10-1571-2016
Raleigh, M., Lundquist, J., and Clark, M. (2015). Exploring the impact of forcing error characteristics on physically based snow simulations within a global sensitivity analysis framework. Hydrol. Earth Syst. Sci. 19, 3153–3179. doi: 10.5194/hess-19-3153-2015
Rasmussen, R., Baker, B., Kochendorfer, J., Meyers, T., Landolt, S., Fischer, A. P., et al. (2012). How well are we measuring snow: the NOAA/FAA/NCAR winter precipitation test bed. Bull. Am. Met. Soc. 93, 811–829. doi: 10.1175/BAMS-D-11-00052.1
Rasmussen, R., Liu, C., Ikeda, K., Gochis, D., Yates, D., Chen, F., et al. (2011). High-resolution coupled climate runoff simulations of seasonal snowfall over colorado: a process study of current and warmer climate. J. Clim. 24, 3015–3048. doi: 10.1175/2010JCLI3985.1
Réveillet, M., Six, D., Vincent, C., Rabatel, A., Dumont, M., Lafaysse, M., et al. (2018). Relative performance of empirical and physical models in assessing the seasonal and annual glacier surface mass balance of Saint-Sorlin glacier (French Alps). Cryosphere 12:1367. doi: 10.5194/tc-12-1367-2018
Réveillet, M., Vincent, C., Six, D., and Rabatel, A. (2017). Which empirical model is best suited to simulate glacier mass balances? J. Glaciol. 63, 39–54. doi: 10.1017/jog.2016.110
Revuelto, J., Lecourt, G., Lafaysse, M., Zin, I., Charrois, L., Vionnet, V., et al. (2018). Multi-criteria evaluation of snowpack simulations in complex alpine terrain using satellite and in situ observations. Remote Sens. 10:1171. doi: 10.3390/rs10081171
Revuelto, J., Vionnet, V., López-Moreno, J.-I., Lafaysse, M., and Morin, S. (2016). Combining snowpack modeling and terrestrial laser scanner observations improves the simulation of small scale snow dynamics. J. Hydrol. 533, 291–307. doi: 10.1016/j.jhydrol.2015.12.015
Riggs, G. A., Hall, D. K., and Román, M. O. (2017). Overview of NASA's MODIS and visible infrared imaging radiometer suite (VIIRS) snow-cover earth system data records. Earth Syst. Sci. Data 9, 765–777. doi: 10.5194/essd-9-765-2017
Roe, G. H. (2005). Orographic precipitation. Annu. Rev. Earth Planet. Sci. 33, 645–671. doi: 10.1146/annurev.earth.33.092203.122541
Roth, A., Hock, R., Schuler, T. V., Bieniek, P. A., Pelto, M., and Aschwanden, A. (2018). Modeling winter precipitation over the Juneau Icefield, Alaska, using a linear model of orographic precipitation. Front. Earth Sci. 6:20. doi: 10.3389/feart.2018.00020
Salomonson, V. V., and Appel, I. (2004). Estimating fractional snow cover from MODIS using the normalized difference snow index. Rem. Sens. Environ. 89, 351–360. doi: 10.1016/j.rse.2003.10.016
Schirmer, M., and Jamieson, B. (2015). Verification of analysed and forecasted winter precipitation in complex terrain. Cryosphere 9, 587–601. doi: 10.5194/tc-9-587-2015
Schlögl, S., Marty, C., Bavay, M., and Lehning, M. (2016). Sensitivity of Alpine3D modeled snow cover to modifications in DEM resolution, station coverage and meteorological input quantities. Environ. Modell. Softw. 83, 387–396. doi: 10.1016/j.envsoft.2016.02.017
Schweizer, J., Jamieson, J. B., and Schneebeli, M. (2003). Snow avalanche formation. Rev. Geophys. 41:1016. doi: 10.1029/2002RG000123
Seity, Y., Brousseau, P., Malardel, S., Hello, G., Bénard, P., Bouttier, F., et al. (2011). The AROME-France convective-scale operational model. Mon. Wea. Rev. 139, 976–991. doi: 10.1175/2010MWR3425.1
Silverman, N., Maneta, M., Chen, S.-H., and Harper, J. (2013). Dynamically downscaled winter precipitation over complex terrain of the Central Rockies of Western Montana, USA. Water Resour. Res. 49, 458–470. doi: 10.1029/2012WR012874
Six, D., and Vincent, C. (2014). Sensitivity of mass balance and equilibrium-line altitude to climate change in the French alps. J. Glaciol. 60, 867–878. doi: 10.3189/2014JoG14J014
Skamarock, W. C., and Klemp, J. B. (2008). A time-split nonhydrostatic atmospheric model for weather research and forecasting applications. J. Comput. Phys. 227, 3465–3485. doi: 10.1016/j.jcp.2007.01.037
Soci, C., Bazile, E., Besson, F., and Landelius, T. (2016). High-resolution precipitation re-analysis system for climatological purposes. Tellus A 68:29879. doi: 10.3402/tellusa.v68.29879
Sold, L., Huss, M., Hoelzle, M., Andereggen, H., Joerg, P. C., and Zemp, M. (2013). Methodological approaches to infer end-of-winter snow distribution on alpine glaciers. J. Glaciol. 59, 1047–1059. doi: 10.3189/2013JoG13J015
Thibert, E., Blanc, R., Vincent, C., and Eckert, N. (2008). Glaciological and volumetric mass-balance measurements: error analysis over 51 years for Glacier de Sarennes, French Alps. J. Glaciol. 54, 522–532. doi: 10.3189/002214308785837093
Thornton, P. E., Running, S. W., and White, M. A. (1997). Generating surfaces of daily meteorological variables over large regions of complex terrain. J. Hydrol. 190, 214–251. doi: 10.1016/S0022-1694(96)03128-9
Unterstrasser, S., and Zängl, G. (2006). Cooling by melting precipitation in Alpine valleys: an idealized numerical modelling study. Q. J. Roy. Met. Soc. 132, 1489–1508. doi: 10.1256/qj.05.158
Vernay, M., Lafaysse, M., Mérindol, L., Giraud, G., and Morin, S. (2015). Ensemble forecasting of snowpack conditions and avalanche hazard. Cold Reg. Sci. Technol. 120, 251–262. doi: 10.1016/j.coldregions.2015.04.010
Vionnet, V., Bélair, S., Girard, C., and Plante, A. (2015). Wintertime subkilometer numerical forecasts of near-surface variables in the Canadian rocky mountains. Mon. Wea. Rev. 143, 666–686. doi: 10.1175/MWR-D-14-00128.1
Vionnet, V., Brun, E., Morin, S., Boone, A., Faroux, S., Le Moigne, P., et al. (2012). The detailed snowpack scheme Crocus and its implementation in SURFEX v7.2. Geosci. Model. Dev. 5, 773–791. doi: 10.5194/gmd-5-773-2012
Vionnet, V., Dombrowski-Etchevers, I., Lafaysse, M., Quéno, L., Seity, Y., and Bazile, E. (2016). Numerical weather forecasts at kilometer scale in the French Alps: evaluation and application for snowpack modeling. J. Hydrometeorol. 17, 2591–2614. doi: 10.1175/JHM-D-15-0241.1
Vionnet, V., Martin, E., Masson, V., Guyomarc'h, G., Naaim-Bouvet, F., Prokop, A., et al. (2014). Simulation of wind-induced snow transport and sublimation in alpine terrain using a fully coupled snowpack/atmosphere model. Cryosphere 8, 395–415. doi: 10.5194/tc-8-395-2014
Viviroli, D., Dürr, H. H., Messerli, B., Meybeck, M., and Weingartner, R. (2007). Mountains of the world, water towers for humanity: typology, mapping, and global significance. Water Resour. Res. 43:W07447. doi: 10.1029/2006WR005653
Keywords: precipitation, mountain, Crocus snowpack model, seasonal snow, glacier, mass balance
Citation: Vionnet V, Six D, Auger L, Dumont M, Lafaysse M, Quéno L, Réveillet M, Dombrowski-Etchevers I, Thibert E and Vincent C (2019) Sub-kilometer Precipitation Datasets for Snowpack and Glacier Modeling in Alpine Terrain. Front. Earth Sci. 7:182. doi: 10.3389/feart.2019.00182
Received: 26 March 2019; Accepted: 01 July 2019;
Published: 02 August 2019.
Edited by:
Michael Lehning, École Polytechnique Fédérale de Lausanne, SwitzerlandReviewed by:
Ruzica Dadic, Victoria University of Wellington, New ZealandTobias Sauter, University of Erlangen Nuremberg, Germany
Copyright © 2019 Vionnet, Six, Auger, Dumont, Lafaysse, Quéno, Réveillet, Dombrowski-Etchevers, Thibert and Vincent. This is an open-access article distributed under the terms of the Creative Commons Attribution License (CC BY). The use, distribution or reproduction in other forums is permitted, provided the original author(s) and the copyright owner(s) are credited and that the original publication in this journal is cited, in accordance with accepted academic practice. No use, distribution or reproduction is permitted which does not comply with these terms.
*Correspondence: Vincent Vionnet, vincent.vionnet@usask.ca